In today’s fast-paced retail environment, staying ahead of the curve is crucial. Predictive analytics is becoming an indispensable tool for retailers aiming to enhance their decision-making processes and improve customer experiences. By leveraging vast amounts of data, predictive analytics helps retailers anticipate market trends, optimize inventory, and personalize marketing strategies. This approach not only drives operational efficiency but also fosters a deeper connection with customers, ensuring they find what they need when they need it.
Retail giants like Walmart and Amazon are already reaping the benefits of predictive analytics. Walmart uses sophisticated models to streamline its supply chain, ensuring that shelves are stocked with the right products at the right times. Amazon, on the other hand, employs predictive algorithms to offer personalized product recommendations, enhancing the shopping experience and boosting sales. These examples highlight how predictive analytics is transforming retail operations, making them more agile and responsive to consumer demands.
From demand forecasting and customer segmentation to price optimization and fraud detection, predictive analytics offers a multitude of benefits that help retailers stay competitive and thrive in a dynamic market.
The Role of Big Data in Retail
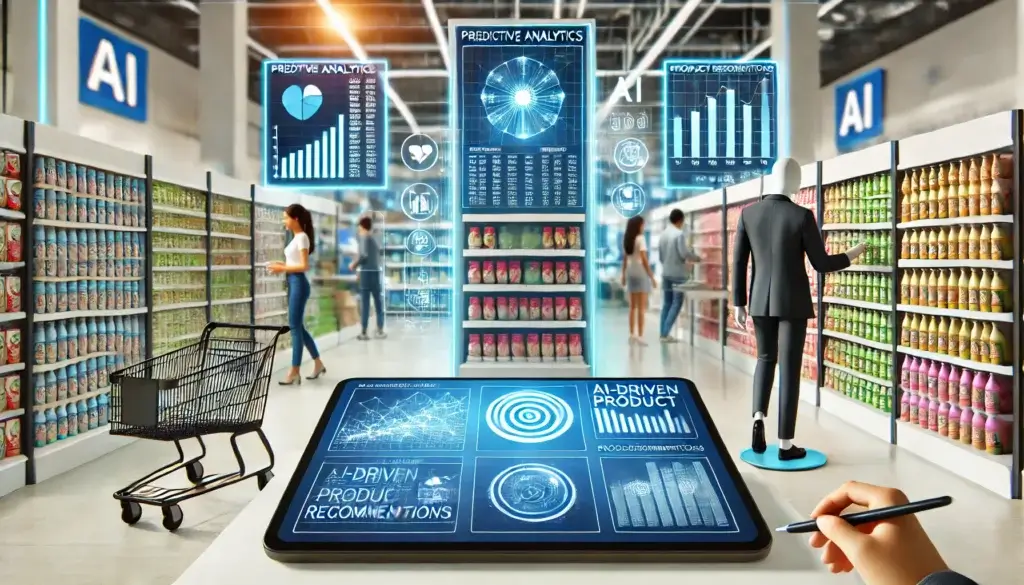
Foundation of Predictive Analytics
Big data is the backbone of predictive analytics in retail. It provides the necessary information and context for understanding customer behaviors and market trends. Without it, predictive models would be incomplete and ineffective.
Importance of Big Data in Predictive Analytics
Big data is crucial because it allows retailers to make informed decisions based on actual consumer behavior and market dynamics. By analyzing large datasets, retailers can uncover patterns and trends that inform everything from inventory management to personalized marketing.
Types of Data Used
Predictive analytics relies on various types of data:
- Customer Transactions: Every purchase made by a customer provides valuable insights into buying habits, preferences, and trends. This data helps retailers understand what products are popular, which items are frequently bought together, and how seasonal changes affect purchasing behavior.
- Social Media Interactions: Social media platforms are a goldmine of customer opinions and preferences. Analyzing likes, shares, comments, and other interactions can reveal customer sentiment and emerging trends. This real-time data helps retailers stay ahead of the curve.
- Market Trends: External data such as economic indicators, competitor pricing, and industry reports help retailers understand the broader market landscape. This context is essential for making strategic decisions that align with current and future market conditions.
Machine Learning and AI’s Role
Machine learning and AI are the engines that drive predictive analytics. These technologies analyze the vast amounts of data collected and identify patterns that humans might miss. Here’s how they contribute:
- Pattern Recognition: Machine learning algorithms can sift through enormous datasets to find patterns and correlations. This helps retailers predict future trends based on historical data.
- Predictive Modeling: AI models use past data to forecast future outcomes. For example, they can predict how changes in pricing will affect sales, or which products are likely to be popular in the upcoming season.
- Real-Time Analysis: AI can process data in real-time, providing retailers with up-to-the-minute insights. This immediacy is crucial for making quick decisions in a fast-paced retail environment.
In essence, big data, combined with the analytical power of machine learning and AI, forms the foundation of predictive analytics in retail. It allows retailers to understand their customers better, optimize their operations, and stay competitive in a rapidly evolving market.
Key Applications of Predictive Analytics in Retail
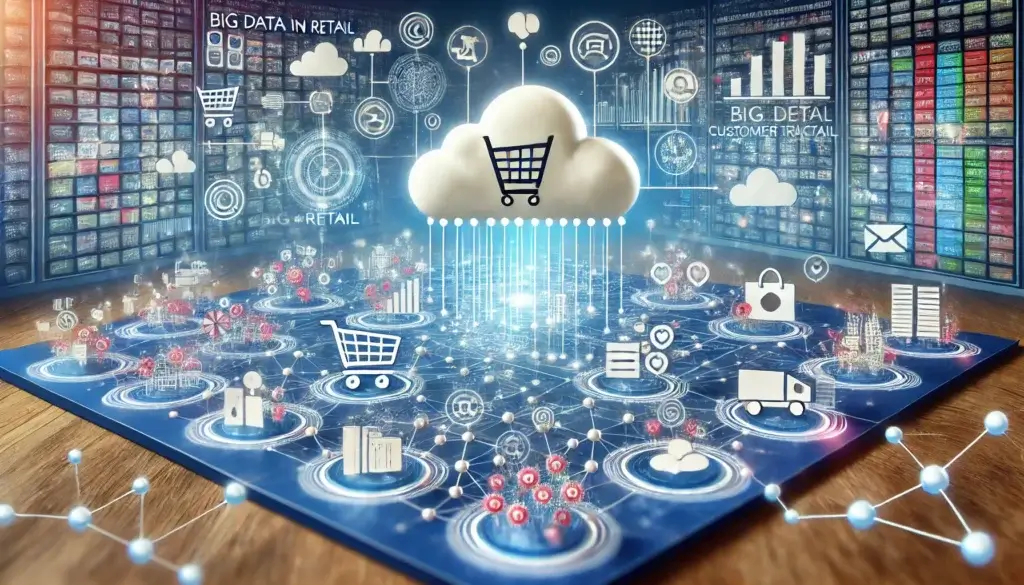
Demand Forecasting
Demand forecasting is a game-changer for retailers. By predicting customer demand for products, retailers can optimize inventory levels, reduce waste, and ensure that popular items are always in stock. This means fewer missed sales opportunities and more satisfied customers.
Case Study: Walmart’s Supply Chain Optimization
Walmart uses predictive analytics to streamline its supply chain operations. By analyzing sales data, weather patterns, and local events, Walmart can forecast product demand with remarkable accuracy. This allows them to maintain optimal inventory levels, ensuring that stores are stocked with the right products at the right time, ultimately improving customer satisfaction and reducing operational costs.
Customer Segmentation
Understanding your customers is key to effective marketing. Predictive analytics helps retailers segment their customer base into distinct groups based on demographics, purchasing behavior, and preferences. This allows for more targeted marketing campaigns and personalized shopping experiences.
Case Study: Target’s Personalized Marketing Efforts
Target employs predictive analytics to enhance its marketing strategies. By analyzing customer data, Target can identify specific segments within its customer base and tailor promotions accordingly. This personalized approach has significantly improved customer engagement and loyalty, demonstrating the power of targeted marketing.
Price Optimization
Price optimization involves using predictive models to set the best possible prices for products. This strategy takes into account competitor pricing, demand elasticity, and customer behavior to maximize revenue and profitability.
Case Study: Amazon’s Personalized Pricing and Promotion Strategies
Amazon leverages predictive analytics to fine-tune its pricing strategies. By analyzing a wealth of data, Amazon can dynamically adjust prices in response to market conditions and customer preferences. This approach not only boosts sales but also enhances the overall shopping experience by offering competitive and personalized pricing.
Inventory Management
Effective inventory management is crucial for minimizing costs and meeting customer demand. Predictive analytics helps retailers forecast product demand, identify slow-moving items, and optimize stock levels to reduce carrying costs and prevent stockouts.
Example: Techniques for Efficient Inventory Management
Retailers use predictive analytics to balance stock levels by forecasting demand and identifying trends. Techniques such as demand sensing and lead time forecasting enable retailers to maintain the right inventory levels, ensuring products are available when customers need them while minimizing excess stock.
Fraud Detection
Fraud detection is essential for protecting both the retailer and the customer. Predictive analytics plays a vital role in identifying and preventing fraudulent activities by analyzing transaction data and detecting anomalies.
Technologies Used: Anomaly Detection Algorithms and User Behavior Analysis
Retailers employ advanced anomaly detection algorithms and user behavior analysis to spot suspicious activities. These technologies can identify patterns that indicate potential fraud, allowing retailers to take proactive measures to prevent financial losses and maintain customer trust.
Predictive analytics is transforming the retail industry by enabling smarter decision-making and enhancing operational efficiency. From demand forecasting to fraud detection, the applications of this technology are vast and impactful, helping retailers stay competitive in an ever-evolving market.
Case Studies
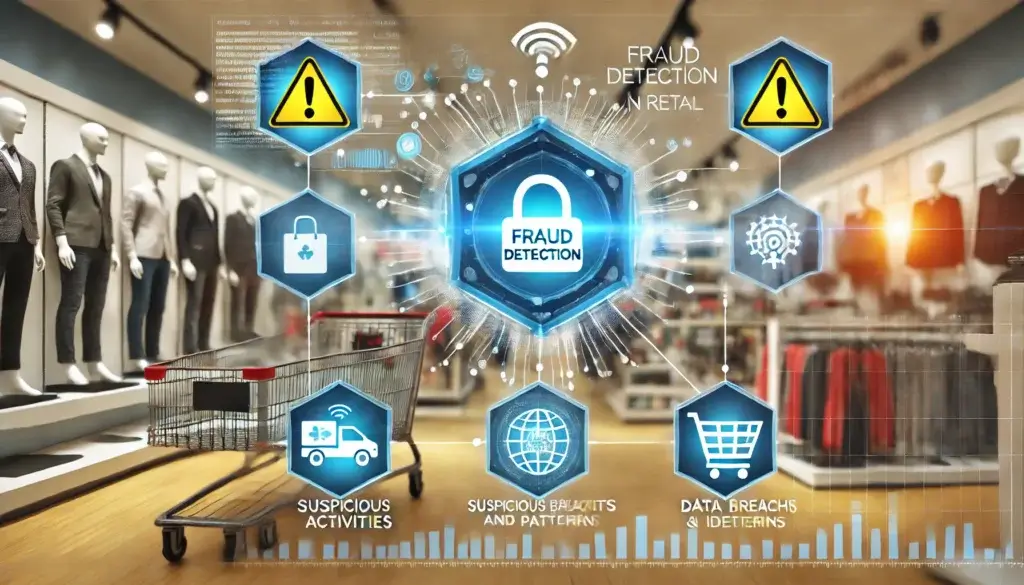
Walmart
Detailed Analysis of Walmart’s Use of Predictive Analytics for Demand Forecasting
Walmart has long been a pioneer in leveraging data to drive its business decisions. Predictive analytics plays a crucial role in Walmart’s demand forecasting efforts. By analyzing vast amounts of data from sales transactions, local events, and weather patterns, Walmart can accurately predict customer demand. This capability allows the company to ensure that its stores are stocked with the right products at the right times, significantly reducing the chances of stockouts and excess inventory.
Impact on Supply Chain Efficiency and Product Availability
Walmart’s use of predictive analytics has had a profound impact on its supply chain efficiency. By accurately forecasting demand, Walmart can optimize its inventory levels and streamline its supply chain operations. This means products are always available when customers need them, enhancing the shopping experience and boosting customer satisfaction. Additionally, the improved efficiency leads to cost savings, as the company can reduce unnecessary inventory and minimize waste.
Amazon
Insight into Amazon’s Recommendation Engine
Amazon’s recommendation engine is one of the most advanced examples of predictive analytics in action. The engine uses collaborative filtering techniques to analyze customer behavior and predict what products a customer might be interested in. By examining purchase history, browsing patterns, and even the buying habits of similar customers, Amazon can provide highly personalized product recommendations.
Benefits of Collaborative Filtering for Up-Sell and Cross-Sell Opportunities
The benefits of Amazon’s recommendation engine are substantial. Collaborative filtering not only enhances the shopping experience by making it easier for customers to find products they might like, but it also drives up-sell and cross-sell opportunities. For example, if a customer is looking at a particular book, the recommendation engine might suggest other books by the same author or related genres. This increases the likelihood of additional purchases, boosting Amazon’s overall sales and revenue.
Target
Exploration of Target’s Customer Segmentation and Personalized Marketing
Target has embraced predictive analytics to refine its customer segmentation and marketing strategies. By analyzing customer data, Target can segment its customer base into distinct groups based on demographics, purchasing behavior, and preferences. This segmentation allows Target to tailor its marketing efforts, delivering personalized promotions and offers that resonate with each customer segment.
Effectiveness in Increasing Customer Loyalty and Marketing ROI
Target’s use of predictive analytics has proven highly effective in increasing customer loyalty and marketing ROI. Personalized marketing efforts result in higher engagement rates, as customers receive promotions and offers that are relevant to their interests and needs. This personalized approach not only drives sales but also builds stronger relationships with customers, fostering loyalty and encouraging repeat business. The improved targeting and relevance of marketing campaigns also lead to better returns on marketing investments, making Target’s marketing efforts more efficient and effective.
Benefits of Predictive Analytics in Retail
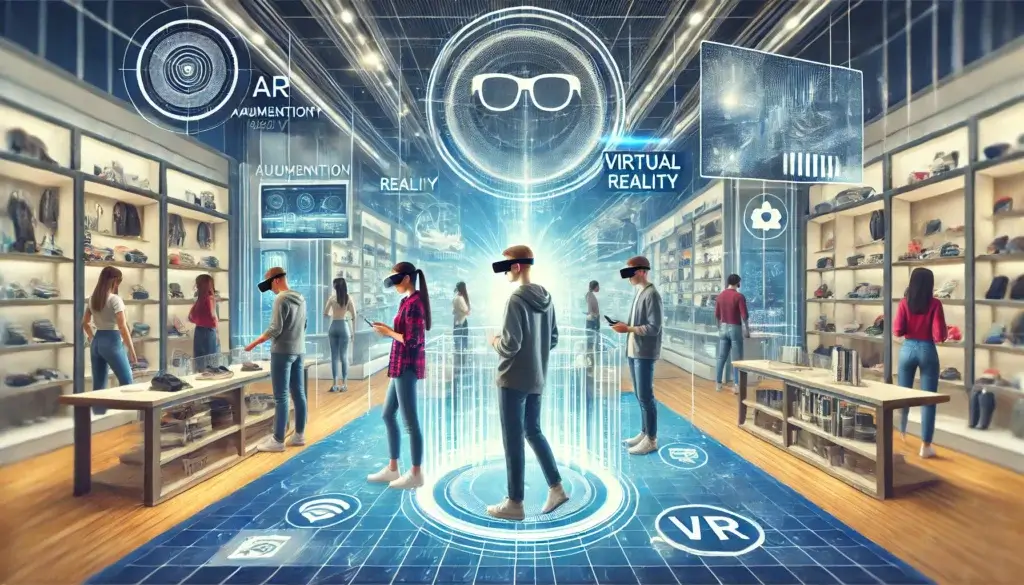
Operational Excellence
Streamlining Operations and Reducing Costs
Predictive analytics is a game-changer for achieving operational excellence in retail. By analyzing vast amounts of data, retailers can streamline their operations and significantly reduce costs. Predictive models help retailers anticipate demand, manage inventory more effectively, and optimize their supply chains. This leads to fewer stockouts, reduced excess inventory, and lower carrying costs. The insights derived from predictive analytics enable better decision-making, allowing retailers to plan more strategically and allocate resources more efficiently.
Examples of Improved Decision-Making and Strategic Planning
For instance, by using predictive analytics, retailers can forecast sales for upcoming seasons based on historical data and market trends. This allows them to adjust their inventory levels accordingly, ensuring that popular items are always in stock while minimizing overstock of less popular items. Additionally, predictive analytics can identify patterns in customer behavior, helping retailers optimize their store layouts, product placements, and staffing levels to enhance operational efficiency and improve the overall shopping experience.
Enhanced Customer Experience
Personalization and Its Impact on Customer Satisfaction
Personalization is at the heart of enhancing customer experience, and predictive analytics makes it possible. By analyzing customer data, retailers can offer personalized recommendations, targeted promotions, and tailored shopping experiences. This level of personalization increases customer satisfaction, as shoppers feel understood and valued. Predictive analytics also enables retailers to anticipate customer needs and preferences, ensuring that they are always one step ahead.
Real-World Benefits Seen in Improved Customer Engagement and Sales
In real-world applications, personalized marketing campaigns driven by predictive analytics have led to higher engagement rates and increased sales. Customers who receive relevant recommendations and offers are more likely to make purchases and remain loyal to the brand. Retailers can also use predictive analytics to improve their loyalty programs, identifying the most valuable customers and offering them exclusive rewards and experiences. This not only boosts customer retention but also drives word-of-mouth referrals and positive brand perception.
Competitive Advantage
Staying Ahead in a Dynamic Market
In today’s rapidly changing retail landscape, staying competitive requires agility and foresight. Predictive analytics gives retailers a strategic edge by providing insights that enable them to adapt quickly to market changes. By forecasting trends and identifying emerging opportunities, retailers can stay ahead of their competitors and capture market share. Predictive analytics also helps retailers optimize their pricing strategies, ensuring they remain competitive while maximizing profitability.
Examples of How Predictive Analytics Provides Retailers with a Strategic Edge
Retailers using predictive analytics can better understand their customers and market dynamics, allowing them to make data-driven decisions that enhance their competitive positioning. For example, by analyzing customer feedback and sales data, retailers can identify gaps in their product offerings and introduce new products that meet customer needs. They can also adjust their marketing strategies in real-time, responding to market trends and customer preferences more effectively than their competitors. This proactive approach helps retailers not only survive but thrive in a dynamic market environment.
Predictive analytics is transforming the retail industry by enabling operational excellence, enhancing customer experiences, and providing a competitive advantage. Retailers who harness the power of predictive analytics are well-equipped to navigate the challenges of the modern market and achieve long-term success.
Implementation Strategies
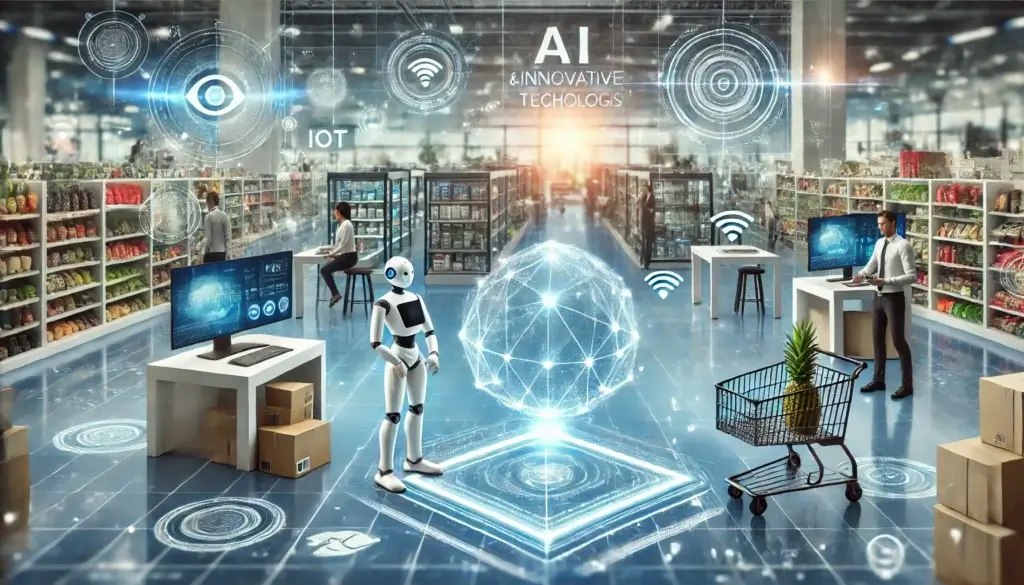
Steps to Leveraging Predictive Analytics
Implementing predictive analytics in retail can seem daunting, but breaking it down into clear steps makes the process manageable and effective. Here’s a straightforward guide to help retailers get started.
Data Collection and Integration from Multiple Sources
The first step in leveraging predictive analytics is gathering data from various sources. This includes customer transactions, social media interactions, inventory records, and market trends. Integrating this data provides a comprehensive view of your business operations and customer behavior. For example, combining point-of-sale data with social media insights can reveal not only what customers are buying but also what they are saying about your products.
Data Cleaning, Feature Selection, and Model Development
Once data is collected, it needs to be cleaned and prepared for analysis. This involves removing duplicates, correcting errors, and standardizing formats to ensure accuracy and consistency. Next, identify the relevant variables or features that will be used in your predictive models. This step might include creating new variables or transforming existing ones to improve predictive accuracy.
After preparing the data, develop predictive models using machine learning or AI algorithms. These models should be evaluated for performance to ensure they provide accurate and reliable predictions. Tools like regression analysis, decision trees, and neural networks can be employed to build these models.
Continuous Monitoring and Optimization of Predictive Models
Predictive analytics is not a set-it-and-forget-it solution. Continuous monitoring and optimization are crucial for maintaining the accuracy and relevance of your models. Regularly update your data and retrain your models to reflect changes in customer behavior, market conditions, and business objectives. This ongoing process ensures that your predictive analytics initiatives remain aligned with your evolving business needs.
Implementing predictive analytics effectively requires careful planning and execution. By following these steps, retailers can harness the power of data to drive better decisions, improve customer experiences, and achieve a competitive edge in the market.
Challenges and Future Trends
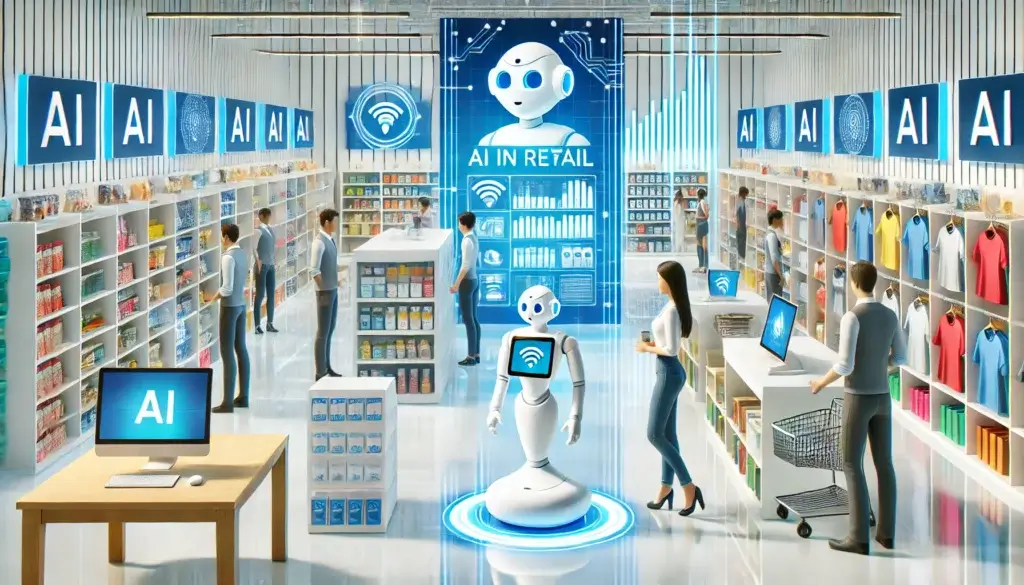
Common Challenges
Data Quality and Integration Issues
One of the biggest hurdles in implementing predictive analytics is ensuring data quality. Retailers often deal with data from multiple sources, which can lead to inconsistencies and errors. Poor data quality can significantly affect the accuracy of predictive models, leading to misguided decisions. Additionally, integrating data from various systems—like point-of-sale, online transactions, and social media—can be complex and time-consuming. Ensuring that all data sources are harmonized and error-free is critical for reliable analytics.
Ensuring Accuracy and Scalability of Predictive Models
Another major challenge is maintaining the accuracy and scalability of predictive models. As business environments and consumer behaviors change, models can quickly become outdated. Retailers must continuously update and refine their models to keep them relevant. This requires ongoing investment in technology and expertise. Furthermore, as the volume of data grows, ensuring that models can scale to handle larger datasets without compromising performance becomes increasingly important.
Future Trends
Emerging Technologies and Their Potential Impact
The future of predictive analytics in retail looks promising, thanks to several emerging technologies. Artificial intelligence and machine learning continue to evolve, offering more sophisticated algorithms that can handle complex data patterns and provide deeper insights. Technologies like natural language processing (NLP) and computer vision are expanding the capabilities of predictive analytics beyond traditional data points, allowing retailers to analyze customer reviews, social media posts, and even video feeds for insights.
Another exciting development is the rise of edge computing, which allows data processing to occur closer to the source of data generation. This reduces latency and enables real-time analytics, making it possible for retailers to react instantly to changing conditions and customer behaviors.
Predictions for the Future of Retail Analytics and Upcoming Innovations
Looking ahead, the integration of predictive analytics with Internet of Things (IoT) devices is expected to revolutionize retail operations. Smart shelves, connected inventory systems, and personalized in-store experiences powered by IoT data will become more common. These innovations will provide retailers with real-time insights into customer preferences and inventory levels, enhancing operational efficiency and customer satisfaction.
Moreover, as consumers become more comfortable with digital interactions, augmented reality (AR) and virtual reality (VR) will play a larger role in retail. Predictive analytics will be used to tailor AR/VR experiences to individual preferences, creating immersive and personalized shopping journeys.
Blockchain technology also holds potential for enhancing predictive analytics by ensuring data integrity and security. With blockchain, retailers can trust the accuracy and authenticity of the data used in their predictive models, leading to more reliable insights and decisions.
In summary, while there are significant challenges in implementing predictive analytics in retail, the future is bright with emerging technologies and innovative applications on the horizon. Retailers that invest in overcoming current obstacles and embracing future trends will be well-positioned to thrive in the competitive landscape.
Conclusion
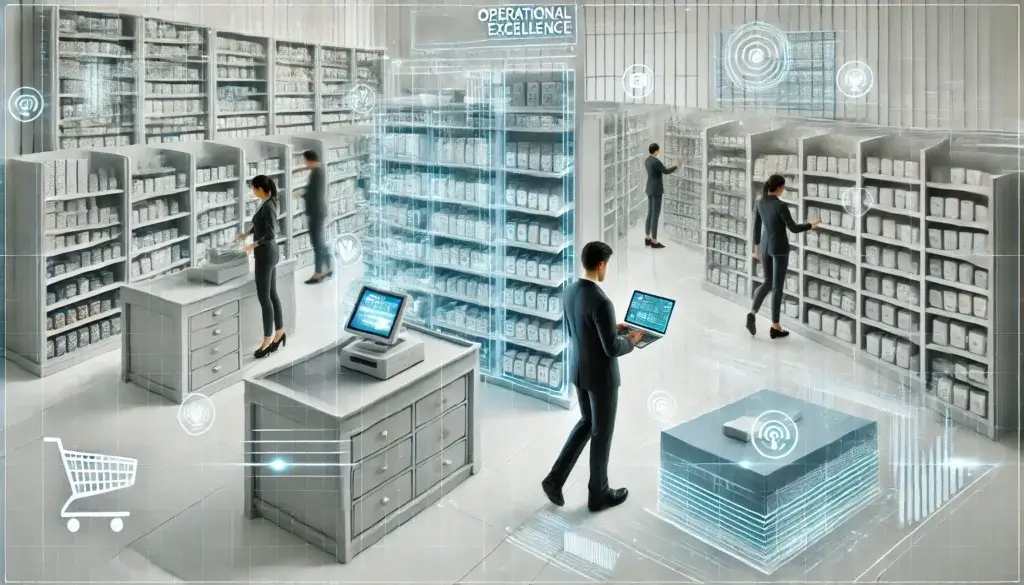
Predictive analytics is transforming the retail industry, providing retailers with the tools they need to optimize operations, enhance customer experiences, and maintain a competitive edge. By leveraging big data, machine learning, and AI, retailers can forecast demand, segment customers, optimize pricing, manage inventory, and detect fraud more effectively.
Case studies from industry leaders like Walmart, Amazon, and Target demonstrate the real-world benefits of predictive analytics. Walmart’s efficient supply chain management, Amazon’s personalized recommendations, and Target’s tailored marketing efforts highlight the power of predictive analytics in driving business success.
However, implementing predictive analytics comes with challenges such as ensuring data quality and model accuracy. As the retail landscape evolves, emerging technologies like AI, IoT, AR, VR, and blockchain promise to further enhance the capabilities of predictive analytics, offering exciting opportunities for innovation and growth.
Retailers who invest in predictive analytics and stay abreast of technological advancements will be well-positioned to thrive in the competitive and dynamic market. By embracing these strategies, they can make smarter decisions, deliver superior customer experiences, and achieve long-term success.
References
- Predictive Analytics: Forecasting Future Trends in Retail
- Retail Insider
- retail-insider.com
- Retail Predictive Analytics: Usage, Benefits & More
- Leafio
- leafio.ai
- To Predict Consumer Behavior, Retailers Turn to Data Analytics
- BizTech Magazine
- biztechmagazine.com
- Predictive Analytics in Retail: Retail Management Research Report
- Coresight Research
- coresight.com
- Predictive Analytics in Retail – Examples and Strategies
- TruRating
- trurating.com
- 10 Ways Predictive Analytics in Retail is Becoming Game Changer
- Matellio Inc
- matellio.com
- Predictive Analytics in Retail Industry: Visualize, Analyze and Decide
- Indian Retailer
- indianretailer.com
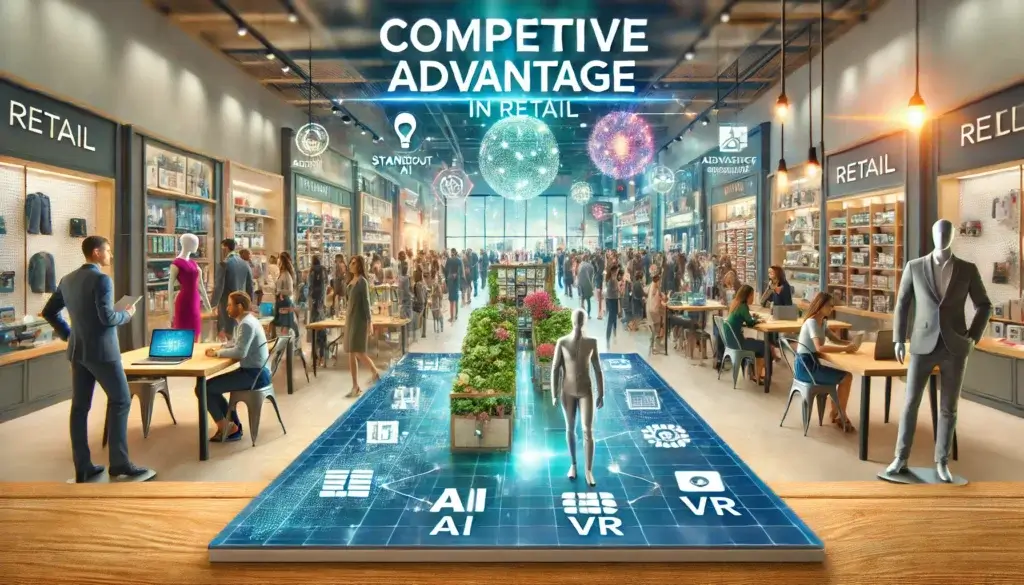