Sales analysis is like the compass for your business, guiding you through the vast sea of market competition and customer preferences. It’s about looking closely at your sales data to understand what’s working and what’s not. This insight helps you tweak your strategies, focus on what customers love, and sell more effectively.
At its heart, sales analysis is about making intelligent, informed decisions. By tracking and examining your sales, you can spot trends, determine which products are hot sellers, and identify which areas might need a boost. It’s also a great way to get to know your customers better, understanding what they buy and why.
In short, sales analysis is essential for any business aiming to grow and stay competitive. It turns your data into actionable insights, helping you steer your company toward success with confidence.
For deeper insights, Salesken.ai and Marketing Tutor offer comprehensive perspectives on the transformative power of sales analysis.
Identifying Sales Analysis Goals and Questions
The primary step in sales analysis is establishing clear and precise objectives. Why is this so crucial? Well-defined goals ensure your analysis is focused, actionable, and tailored to address specific business needs. By articulating what you aim to achieve, you can steer your analysis in the right direction, ensuring every bit of data scrutinized contributes to informed decision-making and strategic planning.
So, how do you formulate the right questions to guide your sales analysis journey? Start by pinpointing the exact issues or opportunities you want to explore. Whether you’re understanding customer behavior, evaluating product performance, or gauging sales team efficacy, your questions should zero in on these focal points. Remember, the sharper your questions, the more insightful your findings.
Here are some exemplary questions to spark your sales analysis curiosity:
- Product Sales Analysis: Which products are flying off the shelves, and why? This question digs into your product lineup’s success rates, uncovering which items resonate with customers and why they’re popular.
- Sales Effectiveness Analysis: How well is your sales strategy converting leads into customers? This inquiry assesses your sales process’s potency, spotlighting areas ripe for optimization.
- Diagnostic Analysis: When sales trends take an unexpected turn, ask why. Identifying the root causes of anomalies can provide critical insights for corrective action.
- Prescriptive Analysis: Faced with sales challenges, what strategic moves should you make? This question seeks actionable solutions to improve sales outcomes.
- Market Research: Who is buying your products, and what are their pain points and preferences? By understanding your audience, you can tailor your offerings and marketing strategies more effectively.
In sum, setting your sales analysis goals and asking the right questions is like charting a map for a treasure hunt; it guides you through the data maze to uncover valuable insights that can propel your business forward. As you embark on this analytical expedition, remember that clarity, relevance, and specificity in your objectives and questions are your best allies in extracting meaningful and actionable intelligence from your sales data.
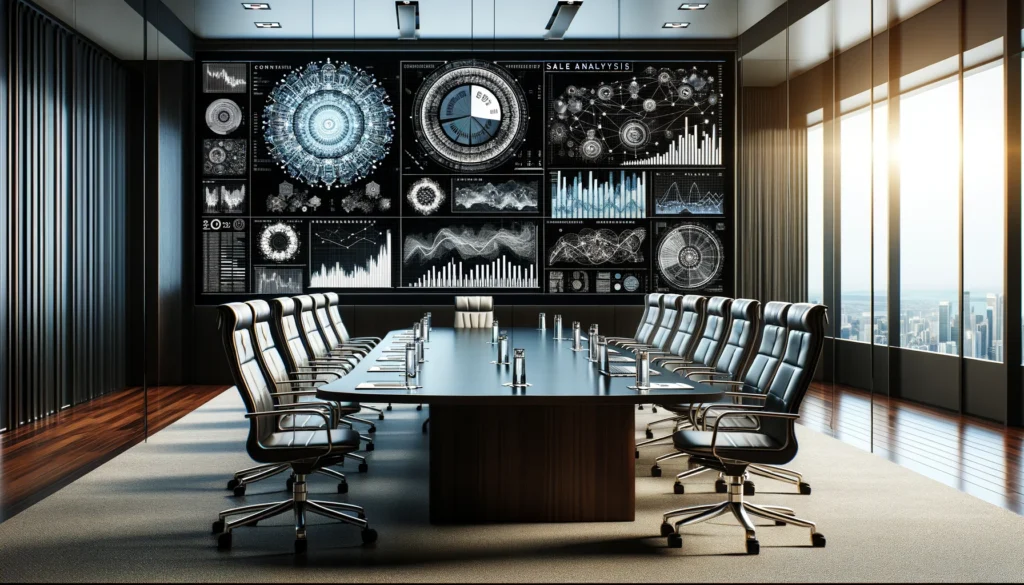
Data Collection
Collecting the right types of sales data is crucial for informed decision-making and strategic planning in sales analysis. Various types of sales data should be collected to offer a comprehensive view:
- Identity Data: This includes basic information about your customers or leads, such as names, addresses, email addresses, phone numbers, and social media profiles.
- Descriptive Data: These details provide a deeper understanding of your customers or leads, including their job titles, industries, interests, and personal preferences.
- Quantitative Data: This numeric data helps you measure and track customer interactions with your company, like purchase history, website visits, engagement levels, and service tickets.
- Qualitative Data: This type of data gives insights into the perceptions, opinions, and feelings of your customers or leads, often collected through surveys, feedback, and direct communications.
Leveraging a Customer Relationship Management (CRM) system is vital to efficiently collecting these data types. CRMs not only help organize and store vast amounts of data but also ensure that every piece of customer information is accessible and actionable. Integrating your CRM with other systems and tools can further enhance data collection by automating the process and ensuring data consistency across various platforms.
For instance, CRM integrations can pull data from email interactions, social media engagements, customer service tickets, and website activities, providing a holistic view of each customer or lead. This integrated approach allows for better segmentation, personalized communication, and, ultimately, more effective sales and marketing strategies.
Moreover, regular data cleansing and validation are essential to maintain the accuracy and reliability of your CRM data. Ensuring that your data is up-to-date and reliable supports better analysis, forecasting, and strategic decision-making, leading to improved sales performance and business growth.
Businesses can streamline their sales processes by emphasizing CRM usage for data collection, enhancing customer relationships, and driving more targeted and effective sales efforts.
Sources:
- Salesforce: What Is Sales Data? Types & Examples
- Fit Small Business: What Is CRM Data? 4 Types of Data to Store in Your CRM
- HubSpot Blog: 4 Types of Data to Have in Your CRM & How to Structure It
- Ringy: CRM Data Guide: Types, Uses, Best Practices, & Data Structure
- HubSpot Blog: Customer Data: What to Collect and How to Put It to Work at Your Company
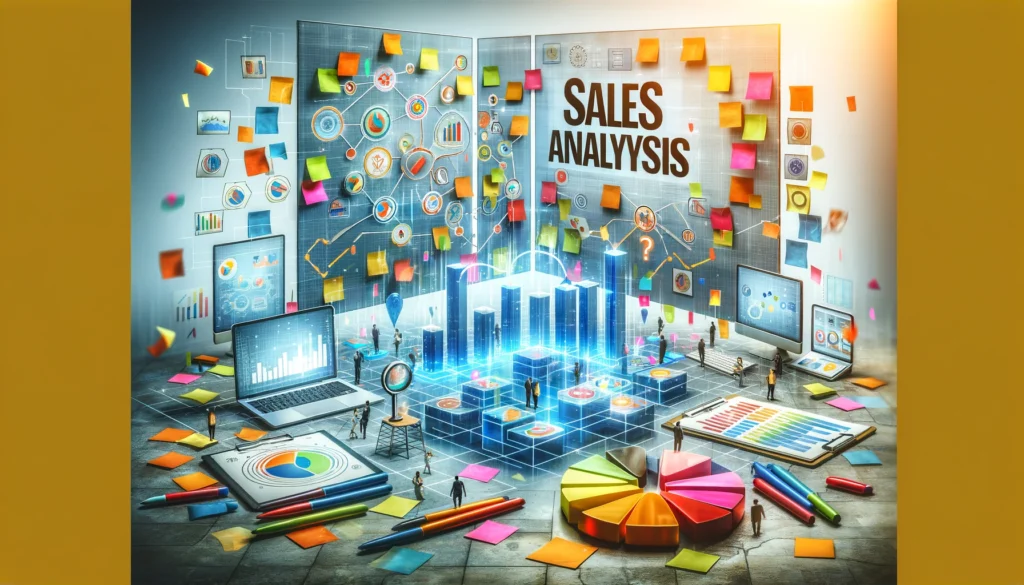
Choosing the Right Sales Analysis Methods
Choosing the suitable sales analysis method is essential to understand various aspects of your business performance, identify growth opportunities, and make informed strategic decisions. Here’s an overview of different types of sales analysis methods and the contexts in which they are most helpful:
- Sales Trend Analysis: This method analyzes historical sales data to identify trends and patterns. It helps businesses understand how sales are evolving and predict future performance. It’s beneficial for spotting seasonal variations, understanding market dynamics, and planning inventory or production levels.
- Sales Performance Analysis: This approach focuses on evaluating the effectiveness of your sales team and strategy by comparing actual sales to set targets. It looks at metrics like conversion rates, average deal size, and sales cycle length. It’s ideal for assessing team performance, identifying areas for training or development, and setting future sales targets.
- Predictive Sales Analysis: Leveraging historical data and statistical algorithms, predictive sales analysis forecasts future sales activities and outcomes. It’s invaluable for strategic planning, budgeting, and anticipating market shifts, enabling businesses to proactively adjust their strategies to capitalize on predicted trends.
- Sales Pipeline Analysis: This method examines the sales pipeline to understand how leads progress through the sales funnel and convert into customers. It identifies bottlenecks and inefficiencies in the sales process, offering insights to optimize lead management and improve conversion rates.
- Product Sales Analysis: By evaluating the performance of different products or services, this analysis helps identify bestsellers, underperformers, and emerging trends. It guides inventory management, product development, and marketing strategies, focusing on customer preferences and market demand.
- Sales Effectiveness Analysis: This analysis measures how well individual sales representatives or teams perform against their goals. It helps identify high and low performers, understand the factors driving success, and devise strategies to improve overall sales effectiveness.
- Diagnostic Analysis: When sales performance deviates from expectations, diagnostic analysis helps identify the underlying reasons. Whether it’s market changes, competitive actions, or internal issues, this analysis provides the insights needed to address challenges and improve results.
- Prescriptive Analysis: Beyond predicting what might happen, prescriptive analysis recommends actions to achieve desired outcomes. It uses data to suggest strategies or tactics that can improve sales performance based on predicted scenarios and potential impacts.
- Marketing Research: Although not strictly a sales analysis, marketing research plays a critical role in understanding the market environment, customer preferences, and competitive landscape. It informs sales strategies by providing a deep understanding of target customers, their needs, and how they perceive your offerings compared to competitors.
By selecting the appropriate sales analysis method for your specific goals and context, you can gain valuable insights to drive revenue growth, improve sales strategies, and enhance overall business performance. Whether you’re looking to understand past trends, forecast future performance, optimize your sales pipeline, or refine your product offerings, there’s a sales analysis technique that can help illuminate the path forward.
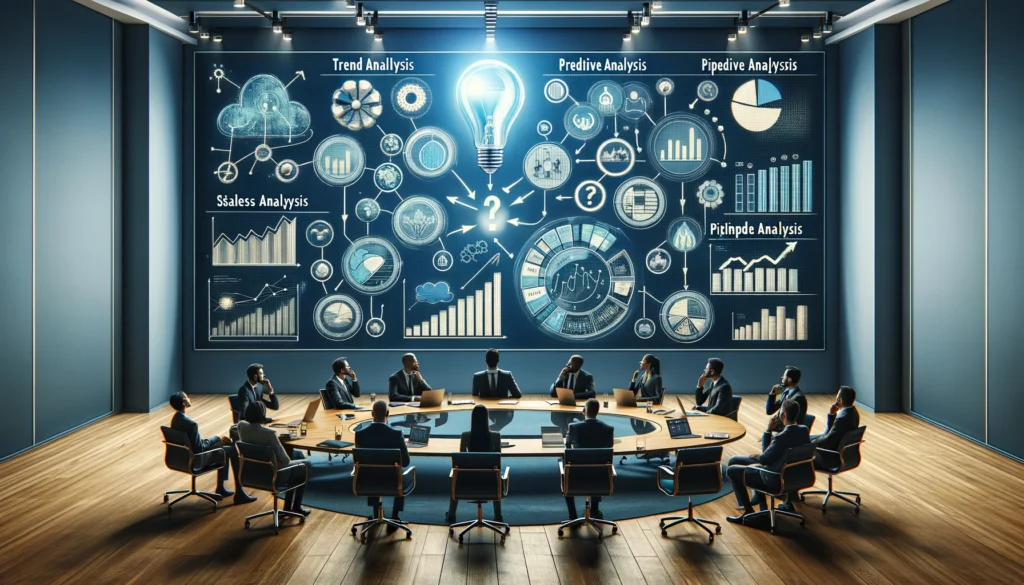
Analyzing Sales Data
Analyzing sales data is essential for understanding the performance of your business, identifying opportunities for improvement, and making informed decisions. Here’s a structured approach to dissecting your sales data effectively:
Step 1: Define Your Objectives
Before diving into the data, it’s crucial to establish what you want to achieve with the analysis. Your objectives will guide the selection of data and metrics relevant to your analysis.
Step 2: Collect and Organize Your Data
Gather sales data from various sources, such as CRM systems, sales reports, and accounting software. Organize this data systematically to facilitate analysis.
Step 3: Identify Key Metrics
Select metrics that align with your objectives. Commonly analyzed sales metrics include conversion rate, average deal size, sales growth, customer acquisition cost (CAC), customer lifetime value (CLV), and churn rate.
Step 4: Calculate Key Sales Metrics
- Conversion Rate: Measure the efficiency of your sales funnel. Calculation: (Number of Conversions / Total Number of Leads) × 100%
- Average Deal Size: Understand the average revenue per sale. Calculation: Total Revenue / Number of Deals Closed
- Sales Growth: Identify trends in sales over time. Calculation: [(Current Period Sales – Previous Period Sales) / Previous Period Sales] × 100%
- CAC: Assess the cost-effectiveness of acquiring new customers. Calculation: Total Costs of Acquiring Customers / Number of New Customers
- CLV: Estimate the total revenue a customer generates. Calculation: Average Purchase Value × Number of Transactions × Average Customer Lifespan
Step 5: Analyze and Interpret the Data
Examine the calculated metrics to uncover trends, patterns, and anomalies. Use this analysis to gain insights into sales performance, customer behavior, and market trends.
Step 6: Derive Actionable Insights
Translate your findings into actionable insights. Identify areas where performance meets or exceeds expectations and areas needing improvement. Formulate strategies to capitalize on strengths and address weaknesses.
Step 7: Implement Changes and Monitor Progress
Apply the insights to refine your sales strategies, processes, and tactics. Continuously monitor key metrics to assess the impact of implemented changes and adjust your strategies as necessary.
By following these steps, you can transform raw sales data into valuable insights that drive informed decision-making and strategic planning, ultimately enhancing your business’s sales performance.
For more detailed guidance, you can explore these resources: Swordfish.ai, Pipedrive, and Small Business Trends, which provide comprehensive insights into sales data analysis processes and best practices.
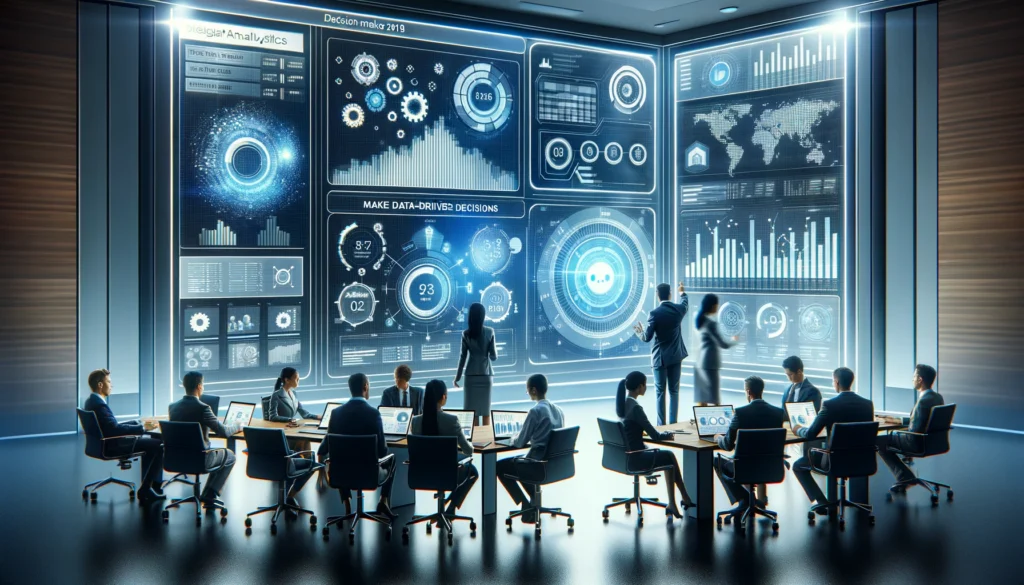
Visualizing and Reporting Findings
Choose the Right Charts and Graphs for the Job
Different types of data and messages require different visualization techniques. Bar charts are excellent for comparing categories; line graphs illustrate trends over time; scatter plots show relationships between variables; and pie charts display part-to-whole relationships. Selecting the right chart type enhances the clarity and impact of your data presentation.
Use Predictable Patterns for Layouts
Leveraging human visual tendencies, such as seeking patterns and organizing information in a logical sequence, can make your data visualization more intuitive. Align your data presentation with common reading patterns (left to right, top to bottom) to ensure a smooth flow of information and easier comprehension.
Implement Clear Color Cues
Color is a powerful tool in data visualization, but its misuse can confuse or mislead. Use color purposefully to highlight key information, indicate relationships, or denote categories. Maintain consistency and limit your palette to prevent visual overload. Proper use of color can quickly convey your message without overwhelming your audience with unnecessary complexity.
Incorporate Contextual Clues
Shapes, icons, or designs related to your subject matter can provide immediate context and enhance understanding at a glance. Using industry-specific symbols or thematic elements can make your visualizations more relatable and memorable.
Tailoring Reports for Different Audiences
Understanding your audience is crucial in data visualization. What works for a data scientist might not resonate with a marketing executive or a general audience. Simplify your visuals and focus on key takeaways for broader audiences, and provide more detailed, complex visualizations for technical or specialist viewers. Clear, audience-tailored visualizations ensure that your message is effectively communicated across different segments of your organization.
Incorporating these best practices into your data visualization and reporting processes will enhance the clarity and impact of your findings and ensure that they are accessible and actionable for all audience segments within your organization.
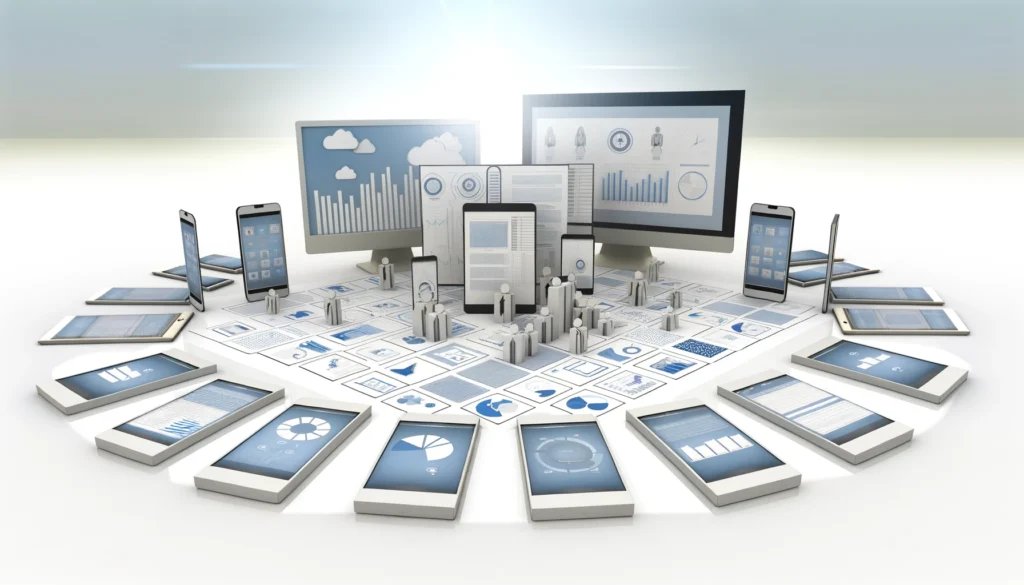
Making Data-Driven Decisions
Utilizing Analysis Insights for Strategic Decision-Making
Data-driven decision-making (DDDM) leverages data to guide business strategies and decisions, enhancing objectivity and reducing the influence of bias. By integrating data analytics, organizations can make informed choices, predict outcomes, and tailor strategies to better meet business objectives and customer needs. For instance, data can reveal customer preferences, market trends, or operational inefficiencies, guiding strategic adjustments and resource allocation.
Case Examples of Effective Data-Driven Sales Strategy Adjustments
- Google: Through Project Oxygen, Google harnessed data from performance reviews and employee retention rates to identify traits of effective managers, improving managerial training programs and significantly boosting favorability scores.
- Starbucks: After facing closures, Starbucks adopted a data-driven approach to selecting new store locations and analyzing demographics and traffic patterns. This analytical method helped Starbucks make informed decisions about where to open new outlets, enhancing its market presence and profitability.
- Amazon: Amazon leverages customer data to drive its recommendation engine, tailoring product suggestions based on user behavior and past purchases, which significantly boosts sales and customer engagement.
- Netflix: By analyzing viewing habits and other user interactions, Netflix has developed a robust recommendation system, greatly enhancing user engagement and retention by offering personalized content suggestions.
- Southwest Airlines: Utilizing customer data, Southwest Airlines could identify the most profitable and popular new services, enhancing customer satisfaction and business growth.
These examples underscore the power of data-driven decisions in refining sales strategies, improving customer experiences, and driving business growth. By embracing data analytics, organizations can make more informed decisions, adapt to market changes, and better meet customer needs, ultimately achieving a competitive edge and enhancing business performance.
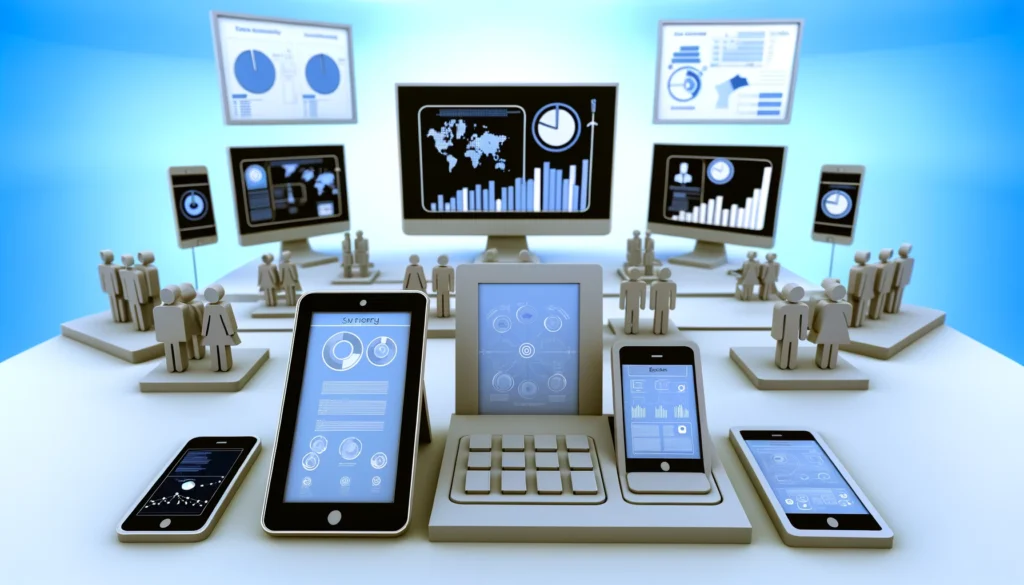
Sales Analysis Tools
Overview of Various Sales Analysis Tools and Software
Sales analysis tools and software are essential for businesses looking to harness data to improve their sales strategies and outcomes. These tools offer a range of functionalities, from predictive analytics to email management and CRM integrations. Here are some noteworthy tools:
- Zoho Zia: An AI-powered assistant that offers predictive insights and automates tasks across various Zoho applications, enhancing productivity and decision-making processes.
- SaneBox: An AI email management tool that prioritizes important emails and organizes your inbox, helping sales teams focus on critical messages.
- ActiveCampaign: Combines email marketing, marketing automation, and CRM, ideal for nurturing and converting leads with insights, task automation, and personalized communications.
- HubSpot Sales Hub: Known for its robust sales analytics features, HubSpot Sales Hub helps track key metrics and maintain customizable dashboards for real-time insights.
- Power BI is a Microsoft tool that offers extensive data visualization options and built-in AI capabilities, scaling from individual salespeople to large enterprises.
- MaxG is a unique B2B sales and recommendation engine that provides intelligent performance insights for marketing efforts and AI-driven actionable suggestions.
- Zoho Analytics is a self-service BI and analytics platform known for its data integration and visualization capabilities. It allows users to create cross-functional reports from varied data sources.
Pros and Cons and Recommendations Based on Business Size and Needs
- Small Businesses: Tools like Zoho Zia, with its comprehensive yet intuitive features, or HubSpot Sales Hub’s free CRM, are excellent choices for smaller businesses needing affordable, versatile solutions.
- Medium-sized Businesses: ActiveCampaign or SaneBox could be more suitable, offering a balance between advanced features and cost-effectiveness, especially in managing customer communications and email marketing.
- Large Enterprises: Power BI or Zoho Analytics would be more appropriate for larger organizations due to their advanced data analysis and integration capabilities, supporting complex, large-scale sales operations.
Each tool has its own pros and cons, so it’s vital to assess your business’s specific needs, budget constraints, and desired outcomes before choosing the correct sales analysis software. By carefully considering these factors, businesses can select the most effective tools to enhance their sales strategies, improve decision-making processes, and ultimately drive better sales results.
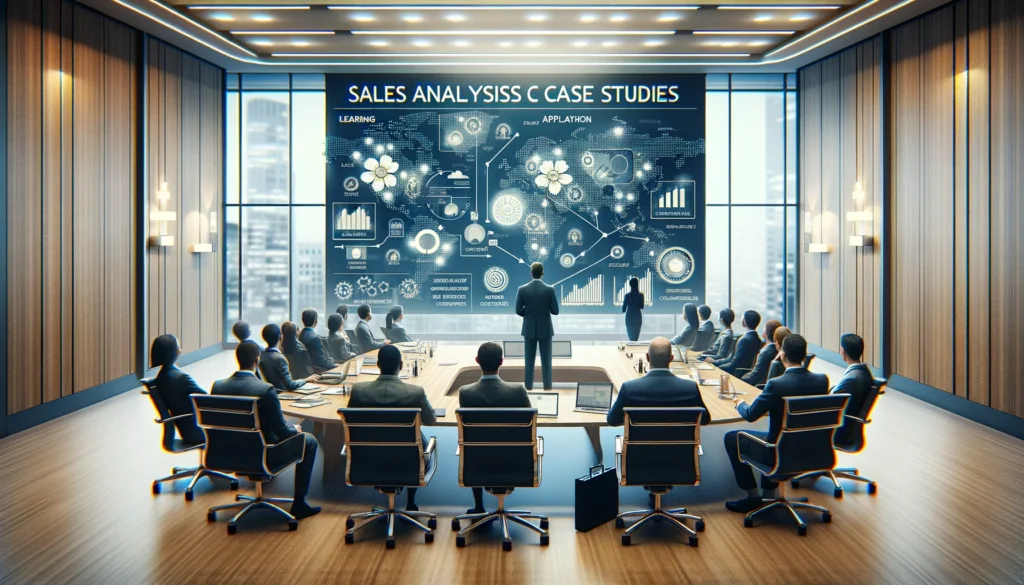
Case Studies and Examples
Exelon’s Data-Driven Audits
Exelon, an energy company, revolutionized its audit process using Tableau for time series analysis. Traditionally, audits were time-intensive and offered limited value. By analyzing an entire year’s data, Exelon identified trends and insights that conventional methods would miss, enhancing the audit’s effectiveness and strategic value.
Stamford Health’s Patient Care Optimization
Stamford Health utilized data analytics to improve patient care and reduce costs. By analyzing data on patient stays, treatments, and outcomes, they optimized treatment times and reduced average patient stays, achieving significant cost savings for both patients and the healthcare system.
Texas Rangers’ Sales Strategy
The Texas Rangers employed time series analysis to enhance ticket sales. They synthesized data across platforms to identify sales opportunities, especially concerning seasonal trends. This proactive approach allowed them to create targeted marketing strategies, significantly impacting ticket sales.
Stitch Fix’s Personalized Styling Service
Stitch Fix leverages big data to tailor its clothing subscription service, employing algorithms to match customer preferences, thereby reducing returns and boosting customer loyalty. This personalized approach has enabled the company to grow its customer base and increase revenues significantly.
UOB’s Risk Management Enhancement
UOB Bank in Singapore harnessed big data for risk management, significantly reducing the time needed for risk calculation and enabling real-time analysis. This transformation has poised UOB for more responsive and informed risk mitigation strategies.
Amazon’s Product Innovation
Amazon uses big data analytics not just for customer service and logistics but also to fuel product innovation and market expansion strategies, exemplified by ventures like Amazon Fresh and Whole Foods. This strategic use of data underpins Amazon’s ability to enter and dominate new markets.
These case studies underscore the transformative power of data analytics across various industries, highlighting the critical role of insightful, data-driven decision-making in achieving business success and innovation.
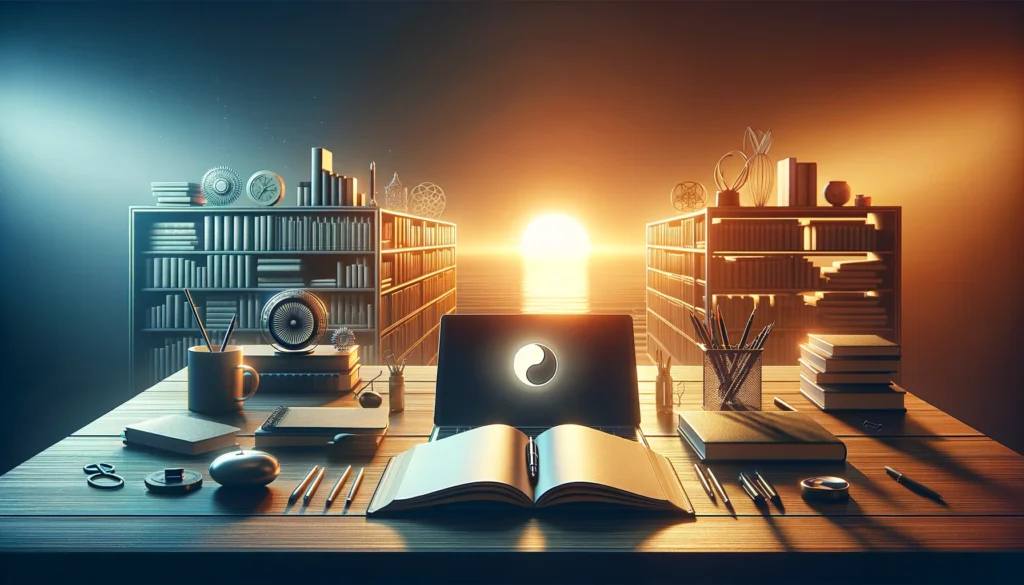
Conclusion
Throughout this guide, we have navigated the various stages of sales analysis, offering a comprehensive roadmap to harness data for informed decision-making. By meticulously dissecting each phase, from setting precise objectives and gathering diverse data to selecting appropriate analysis methods and implementing findings, this guide aims to empower businesses to optimize their sales strategies effectively.
Sales analysis emerges as an indispensable compass, directing businesses through the complexities of market competition and evolving customer preferences. The journey through identifying clear analysis goals, mastering data collection with CRM systems, and choosing the proper analysis techniques unveils the transformative potential of data-driven insights. By delving into detailed methodologies for analyzing sales data and adeptly visualizing and reporting findings, businesses are equipped to make strategic decisions that drive growth and competitiveness.
Moreover, the exploration of diverse sales analysis tools underscores the importance of selecting software that aligns with a company’s size and specific needs, facilitating targeted, efficient sales initiatives. Real-world case studies from Exelon, Stamford Health, the Texas Rangers, Stitch Fix, UOB Bank, and Amazon further illustrate the profound impact of leveraging sales analysis to achieve operational excellence, customer satisfaction, and market innovation.
In conclusion, this guide underscores the pivotal role of sales analysis in crafting a data-informed narrative for your business, enabling a proactive, strategic approach to sales optimization. By embracing the principles and practices outlined herein, businesses can harness the full potential of their sales data, fostering a culture of continuous improvement and data-driven success. As we’ve seen, the journey through sales analysis is not just about understanding past performance but about shaping a future where data-driven decisions illuminate the path to sustained growth and market leadership.
Resources
- “What is Sales Analysis?” from LeadSquared [https://www.leadsquared.com/learn/sales/what-is-sales-analysis/]
- “Sales Analysis” from FineReport [https://www.finereport.com/en/data-analysis/sales-analysis.html]
- “Sales Analysis” from DealHub [https://dealhub.io/glossary/sales-analysis/]
- “Sales Analysis” from EngageBay [https://www.engagebay.com/blog/sales-analysis/]
- “The Ultimate Guide to Sales Analysis Reports” from Databox [https://databox.com/sales-analysis-report]
- “Sales Analysis: Definition, Types, and How to Perform One” from Pipefy [https://www.pipefy.com/blog/sales-analysis/]
- “10 Essential Sales Reports for Sales Leaders” from HubSpot [https://blog.hubspot.com/sales/essential-sales-reports-for-sales-leaders]
- “How to Perform a Sales Analysis (Step-by-Step): Methods & Metrics” from Close [https://blog.close.com/sales-analysis/]