Sales data analysis might sound technical, but at its heart, it’s simply about understanding what’s driving your business. It’s the practice of digging into sales numbers, customer habits, and performance patterns to discover what’s working, what’s not, and what steps could take your business to the next level. Whether you’re just starting out or looking to grow, sales data analysis can give you a clearer, more accurate picture of your business.
So, why is this so important? In today’s fast-paced world, guessing isn’t an option. Data analysis helps you spot opportunities, identify hidden trends, and make smart decisions that actually move the needle. With the right approach, you can understand which products your customers love, figure out where there might be gaps, and adjust your sales strategy based on what the numbers really say. In short, good analysis lets you make choices with confidence and grow sustainably.
In this guide, we’ll break down each step to help you dive into your sales data with ease—from setting clear objectives to gathering and cleaning your data, analyzing it, and visualizing it in a way that’s easy to understand. Whether you’re a complete beginner or just looking to level up your skills, we’ve got you covered. By the end, you’ll have a solid, actionable approach to analyze your sales data effectively.
Understanding Sales Data
When it comes to analyzing sales data, the first step is knowing what kind of data you’re working with and where it’s coming from. Sales data isn’t just about numbers—it’s the story of your business, told through every transaction, interaction, and customer preference. Let’s break down the different types of sales data, the usual sources, and the key metrics that will help you track your business’s performance.
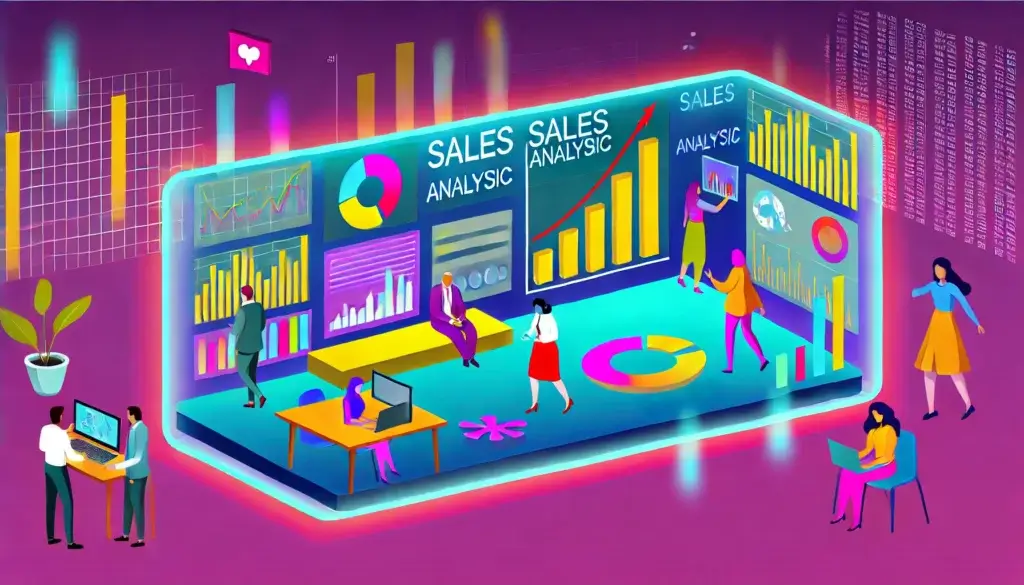
Types of Sales Data
There are a few main types of sales data to get familiar with:
- Transactional Data: This is the bread and butter of sales data. It includes everything related to individual sales transactions—what was sold, when, where, and to whom. Each transaction is a snapshot of a customer’s choice, and when you look at them together, they reveal patterns in product popularity, purchasing frequency, and timing.
- Customer Demographics: Demographic data is all about who your customers are. This can include age, gender, location, job title, and other factors that help you build a profile of your customer base. Understanding who your customers are can help you tailor your sales strategies and target the right audiences.
- Sales Activities: Sales activities refer to all the actions your sales team takes, such as calls, emails, meetings, and other touchpoints with potential or existing customers. Analyzing sales activities can help you understand what actions are leading to sales and where there may be room for improvement.
Sources of Sales Data
So, where do you find this valuable data? Here are some of the most common sources:
- CRM Systems: A CRM (Customer Relationship Management) system is often a goldmine for sales data. It keeps track of every interaction with customers, as well as their purchase history, preferences, and feedback. A good CRM can make it easy to pull data on everything from individual customer journeys to overall sales trends.
- E-commerce Platforms: If you sell online, your e-commerce platform holds a wealth of information about customer purchases, browsing behavior, abandoned carts, and more. This data can be particularly valuable for understanding online shopping trends and optimizing the customer experience.
- POS Systems: For brick-and-mortar businesses, the point-of-sale (POS) system captures data on in-store purchases. This includes details about what’s sold, how much of it, and when—helpful for inventory management and understanding customer demand patterns.
Key Metrics and KPIs to Monitor
Once you have your data, it’s important to know which metrics to focus on. These key performance indicators (KPIs) give you a clear view of how your business is doing:
- Conversion Rate: This is the percentage of leads or potential customers that actually make a purchase. A high conversion rate means your sales efforts are effective, while a low one may indicate issues with your messaging, product offerings, or sales funnel.
- Average Deal Size: This metric tells you the average dollar amount of each sale. It’s a useful indicator of your sales performance and helps you set realistic sales targets. Increasing your average deal size can have a big impact on your bottom line.
- Customer Acquisition Cost (CAC): CAC measures how much it costs to acquire a new customer. It includes expenses on marketing, sales efforts, and any other costs tied to bringing in new business. Lowering your CAC is often a key goal, as it means you’re getting more value from your marketing and sales efforts.
Step 1: Define Objectives
Before diving into the data, it’s essential to know what you’re looking for. Defining clear objectives is the foundation of effective sales data analysis, giving you a sense of direction and ensuring that the insights you uncover are actually useful. Setting solid goals helps you focus your analysis and align it with the bigger picture of your business strategies.
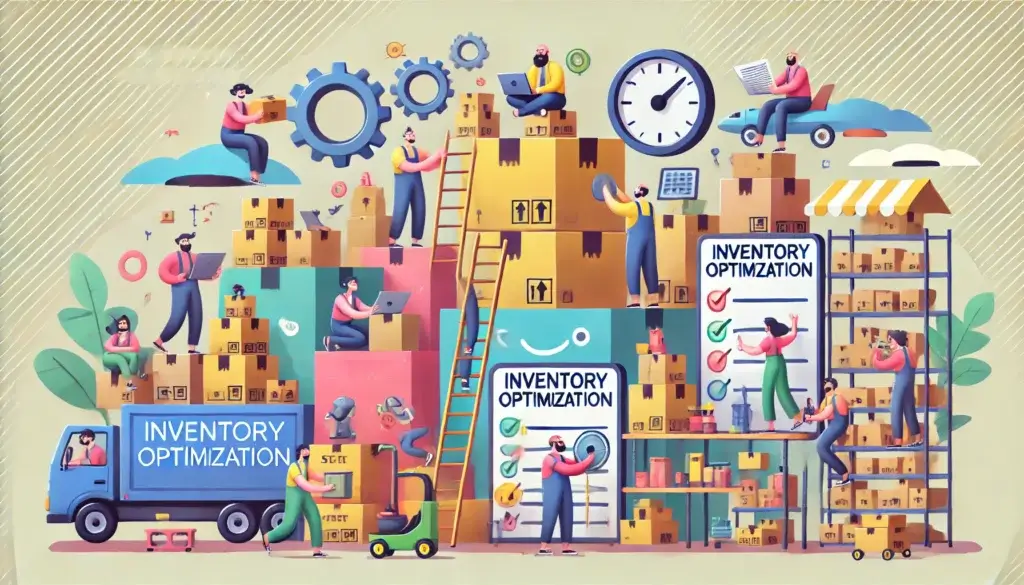
Setting Clear, Measurable Goals for the Analysis
The best objectives are clear and measurable. Instead of vague goals like “boost sales” or “improve customer satisfaction,” try to pinpoint exactly what you want to learn or achieve. A well-defined objective could be something like, “Identify the top five products in terms of sales growth over the last quarter” or “Analyze customer purchase frequency to optimize our email marketing strategy.” By setting goals that are specific and measurable, you’ll know exactly when you’ve hit the mark and can take meaningful action based on your findings.
Aligning Objectives with Business Strategies
Your sales analysis shouldn’t be done in a vacuum; it should tie back to the larger goals and strategies of your business. Take a moment to think about how your analysis can support your company’s overall strategy. For instance, if your business is focused on growing its e-commerce presence, then objectives around understanding online purchasing behavior or improving the online customer journey would be relevant. When your analysis goals align with your business strategy, the insights you gather are much more likely to make a real impact.
Examples of Common Objectives
Not sure where to start? Here are a few common objectives that can bring value to your sales data analysis:
- Identifying Top-Performing Products: Knowing which products are the stars of your lineup can help you focus your marketing and sales efforts more effectively. This type of analysis can reveal seasonal trends, high-demand items, or opportunities to introduce complementary products.
- Understanding Customer Behavior: By analyzing customer purchase patterns, you can get insights into what drives their buying decisions. Are they more likely to buy at certain times of the year, or do they prefer certain product categories? This information can be a game-changer for crafting targeted marketing campaigns or tailoring your product offerings.
- Evaluating Sales Channels: If you’re selling across multiple channels (e.g., online, in-store, third-party marketplaces), it can be valuable to understand which ones are performing best. Knowing where most of your revenue comes from allows you to focus resources on the most profitable channels or work on improving the underperforming ones.
Step 2: Data Collection
Once you’ve set your objectives, it’s time to gather the data that will help you reach them. Data collection might seem like a straightforward step, but knowing where to find the right data and making sure it’s accurate are essential for a successful analysis. Collecting the right data in the right way means you’ll have reliable information to work with, giving you a solid foundation for insights and action.
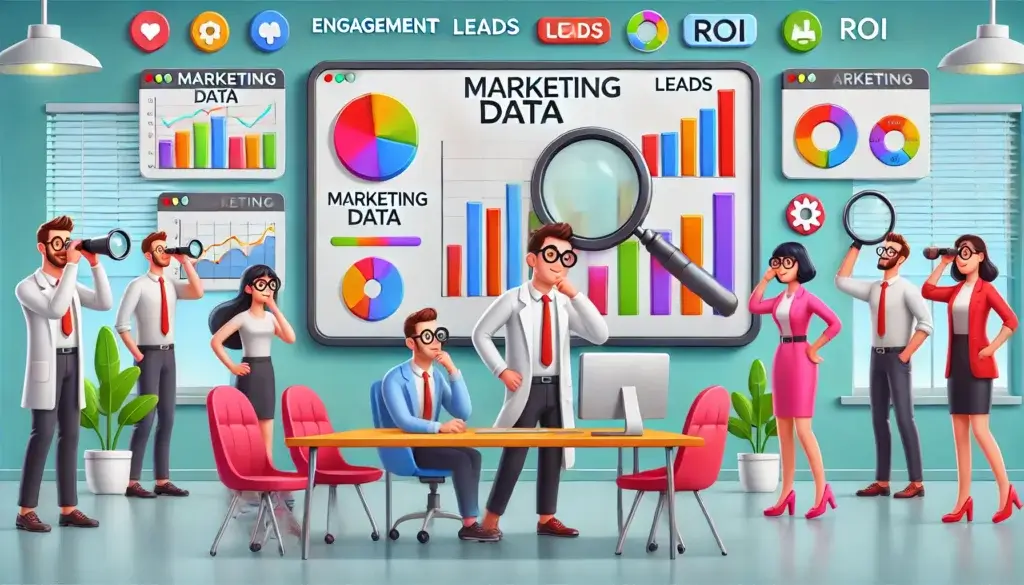
Identifying Relevant Data Sources
The first step in data collection is figuring out where to get the information you need. Your data sources should align with the objectives you set in the previous step. Here are some common sources you might consider:
- CRM Systems: If customer relationships and interactions are part of your objectives, your CRM system is a natural choice. It can give you a comprehensive view of customer behaviors, sales history, and engagement patterns.
- E-commerce Platforms and POS Systems: For businesses with both online and in-store sales, data from e-commerce platforms and point-of-sale (POS) systems is invaluable. These platforms capture transactional data, which can reveal trends in product popularity, peak buying times, and customer preferences.
- Marketing Tools and Web Analytics: If your goals involve understanding how customers are finding your products or where they’re engaging, look into your marketing and analytics tools. Platforms like Google Analytics or social media insights can offer data on user behavior, traffic sources, and engagement.
The right sources for you will depend on your business model and goals, but taking the time to gather data from the most relevant sources will make your analysis much more impactful.
Ensuring Data Quality and Accuracy
Quality data is key. If your data isn’t accurate, up-to-date, or consistent, your analysis can lead to misleading insights. Here are a few ways to make sure your data is in good shape:
- Check for Completeness: Make sure your data sets are complete and free from gaps. Missing information can skew your analysis, so fill in the gaps wherever possible or adjust your approach if certain data is unavailable.
- Ensure Consistency: Data coming from different sources often needs to be standardized. For example, dates might be formatted differently in various systems, or product names might not match exactly. Cleaning up these inconsistencies before you start analyzing will save time and improve accuracy.
- Verify Accuracy: Regularly audit your data for errors or duplicates, especially if it’s collected automatically. Even small errors can add up and affect your findings, so it’s worth checking everything before diving into the analysis.
Tools and Methods for Data Collection
Depending on your needs, there are plenty of tools and methods to help streamline the data collection process:
- Data Integration Platforms: Tools like Zapier, MuleSoft, or Integromat can help you pull data from multiple sources into one place, simplifying the collection process and ensuring you have all relevant information on hand.
- Manual Data Extraction: Sometimes, especially in smaller businesses, manually exporting data from sources like CRM software, POS systems, or spreadsheets can work well. This is especially useful for one-time analysis or when you only need a few data points.
- APIs and Custom Scripts: For more complex data needs, especially if you’re working with large volumes of data, APIs or custom scripts can automate the data collection process. This requires more technical setup but can be powerful for ongoing analysis.
Step 3: Data Cleaning and Preparation
Data cleaning and preparation might not be the most glamorous part of data analysis, but it’s one of the most crucial. Raw data often comes with gaps, inconsistencies, and formatting issues that can cloud your analysis. Taking the time to clean and organize your data ensures you’re working with reliable information, setting the stage for accurate and actionable insights.
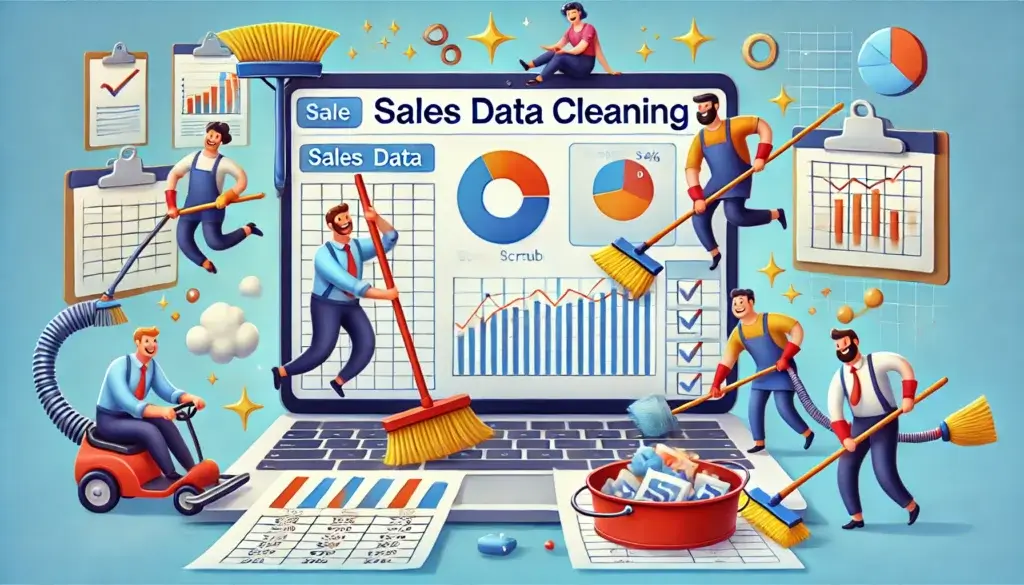
Handling Missing or Inconsistent Data
Missing or inconsistent data is almost inevitable, but it doesn’t have to be a roadblock. Here are a few ways to handle it:
- Fill in the Blanks: If certain fields are blank or incomplete, look for ways to fill them in. For example, if a customer’s email is missing, check other databases or sources for that information. However, don’t force it—sometimes it’s better to leave a gap than to guess.
- Remove Irrelevant Data: If some of your data is incomplete and isn’t critical to your analysis, it might make sense to filter it out. This can help you focus on high-quality data and keep your analysis accurate.
- Use Consistent Terms: Inconsistent data—like “US” versus “United States” or “1/1/22” versus “January 1, 2022”—can lead to confusion. Clean up these inconsistencies by deciding on a standard format for your analysis.
Standardizing Data Formats
Data from different sources often comes in various formats, making it tricky to analyze without a little formatting work. Standardizing your data ensures that everything lines up, making analysis a smoother process.
- Date and Time Formats: Standardize dates and times to a consistent format. For instance, if you’re analyzing sales by day, choose a format like “YYYY-MM-DD” across the board. This makes it easy to spot trends and compare time periods.
- Units of Measure: If you’re working with data that includes units (like prices in different currencies or weights in different measures), convert everything to a single, consistent unit. This allows for accurate comparisons and calculations.
- Naming Conventions: Make sure any names or categories are uniform. For example, if one data source uses “Client” and another uses “Customer,” standardize to one term across your dataset.
Organizing Data for Analysis
Once your data is clean and standardized, it’s time to organize it for easy access and analysis. Organized data helps you see connections and patterns more clearly, making it easier to draw meaningful insights.
- Segment Data by Categories: Organize your data into logical categories that align with your analysis goals. For example, you might segment by region, product type, or customer demographics, depending on what you’re trying to learn.
- Use a Structured Format: Arrange your data in a structured format, like a spreadsheet or a database, where each row represents a unique transaction or customer, and each column holds specific attributes (e.g., date, product, sales amount). This layout makes it easier to filter, sort, and analyze.
- Create a Backup: Always keep a clean copy of your original data as a backup. This way, if you need to go back or re-clean something, you won’t lose any of your work.
Step 4: Data Analysis Techniques
With clean, organized data in hand, you’re ready to start analyzing! Data analysis is all about uncovering the stories hidden in your numbers. There are a few main types of analysis you can use, each serving a different purpose and helping you understand your data from multiple angles. Let’s go over these techniques and how each one can offer insights into your sales performance.
Descriptive Analysis: Summarizing Data to Understand Current Performance
Descriptive analysis is the first step in making sense of your data. Here, you’re simply looking to summarize and understand what’s happened in the past. By calculating averages, totals, and percentages, you can get a clear picture of your current sales performance.
For example, you might look at your total sales for the past quarter, the average purchase value, or the most popular product categories. This type of analysis doesn’t dig into why things happened—it’s about understanding where things stand right now.
Diagnostic Analysis: Identifying Causes of Performance Trends
Once you have a snapshot of your current performance, diagnostic analysis helps you dig deeper and understand why you’re seeing certain trends. This technique looks for patterns and correlations, helping you identify factors that might be affecting your sales.
For instance, if you notice a dip in sales last month, diagnostic analysis can help you pinpoint possible causes. Maybe it aligns with a decrease in marketing spend, a change in pricing, or seasonal factors. This type of analysis helps you uncover the “why” behind your numbers, providing valuable context for your data.
Predictive Analysis: Forecasting Future Sales Trends
Predictive analysis takes your understanding of current and past trends and uses it to make forecasts about the future. This technique relies on historical data to predict future sales patterns, giving you a head start on planning for upcoming months or quarters.
For example, if your data shows an annual uptick in sales every holiday season, predictive analysis can help you estimate how much inventory to stock or how much to allocate to marketing. While it’s not a guarantee of what will happen, predictive analysis can help you make informed predictions and prepare for potential scenarios.
Prescriptive Analysis: Recommending Actions Based on Data Insights
The final step in your analysis journey is prescriptive analysis, which takes all the insights you’ve gathered and translates them into actionable recommendations. This type of analysis suggests specific steps you can take to improve performance, based on what the data reveals.
If your diagnostic and predictive analyses indicate that your best sales come from email campaigns, prescriptive analysis might recommend focusing more resources on email marketing. Or, if certain products are performing well in certain regions, it could suggest adjusting your regional inventory. The goal of prescriptive analysis is to turn insights into strategy, helping you take data-driven action.
By using these different analysis techniques—descriptive, diagnostic, predictive, and prescriptive—you can explore your data from all angles, moving from understanding your current performance to actively planning for the future. Together, these techniques provide a powerful toolkit for making the most of your sales data.
Step 5: Data Visualization
After analyzing your data, the next step is to present your findings in a way that’s easy to understand—and that’s where data visualization comes in. Visualizing your data makes it simpler to spot patterns, compare metrics, and share insights with others. A well-made chart or graph can quickly tell the story behind your numbers, making your data far more impactful than rows of figures alone.
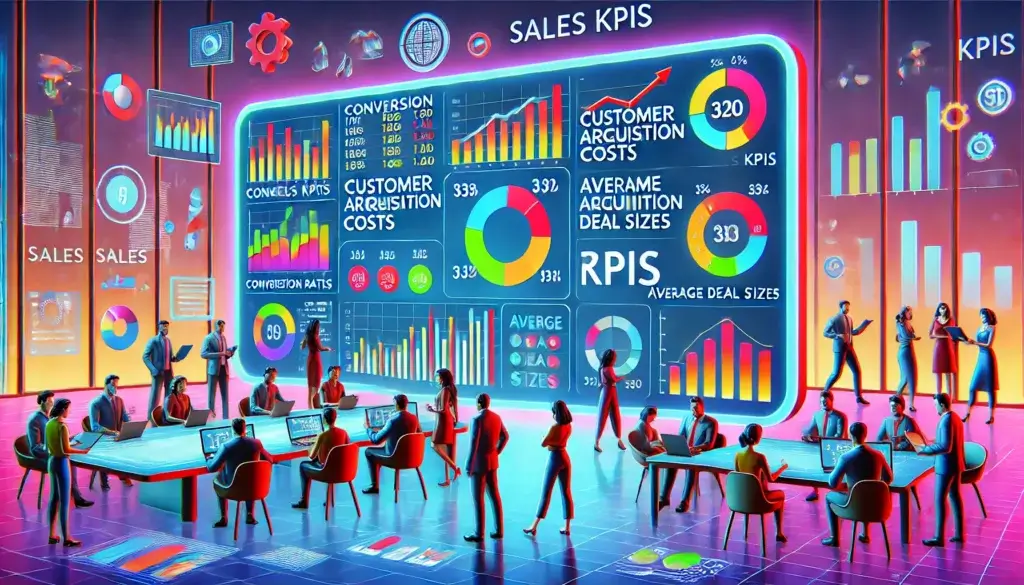
Importance of Visualizing Data for Better Understanding
Visuals bring data to life, helping you and your team understand trends and outliers at a glance. Instead of combing through spreadsheets, you can look at a graph that shows sales growth over time or a pie chart breaking down product performance by category. Visuals make it easier to see the “big picture” and communicate your findings effectively, whether you’re explaining them to stakeholders, team members, or customers.
Common Visualization Tools and Techniques
There are a few popular tools and techniques that can make visualizing data both simple and effective:
- Charts and Graphs: Line charts, bar graphs, and pie charts are classic visuals that work well for showing trends over time, comparing categories, and breaking down proportions. For example, a bar chart is great for comparing sales by product, while a line chart can show how sales have grown month by month.
- Dashboards: If you’re tracking multiple metrics, a dashboard can pull all your visualizations together in one place. Tools like Tableau, Power BI, or Google Data Studio allow you to create custom dashboards, where you can view KPIs, track performance, and get real-time updates in a single view.
- Heatmaps and Geomaps: Heatmaps are useful for identifying areas with high or low activity, such as website clicks or product interest. Geomaps, on the other hand, show data by location, which is helpful for businesses that operate across different regions or countries.
These tools allow you to choose the right type of visualization based on what you want to communicate, making your data easy to interpret and understand.
Best Practices for Creating Effective Visualizations
Good visuals are clear, focused, and tailored to the audience. Here are a few tips to ensure your visualizations are effective:
- Keep It Simple: Avoid cluttering your visuals with too much information. Stick to one main message per chart, and use simple labels and colors to make it easy to read at a glance.
- Use the Right Chart Type: Each chart type has its strengths. Use line charts for trends over time, bar charts for comparisons, and pie charts for proportions. Choosing the right chart type helps your message come across clearly.
- Tell a Story: Let your visuals lead your audience through the data. For example, start with a chart that shows overall sales, then drill down into specific products or regions. This creates a narrative that guides your viewers to key insights.
- Highlight Key Data Points: Draw attention to important numbers by using color, bold text, or annotations. This helps your audience quickly identify the most critical insights in the visualization.
By using these tools and following best practices, you can create visuals that enhance understanding and make your data insights more compelling. A well-crafted visualization not only makes your analysis easier to grasp but also empowers others to take action based on your findings.
Step 6: Interpretation of Results
Now that you’ve visualized your data, it’s time to dig into what it all means. Interpreting your results is about connecting the dots between your findings and your business goals. This step is where you turn raw insights into real-world actions that can help your business grow, improve, and adapt.
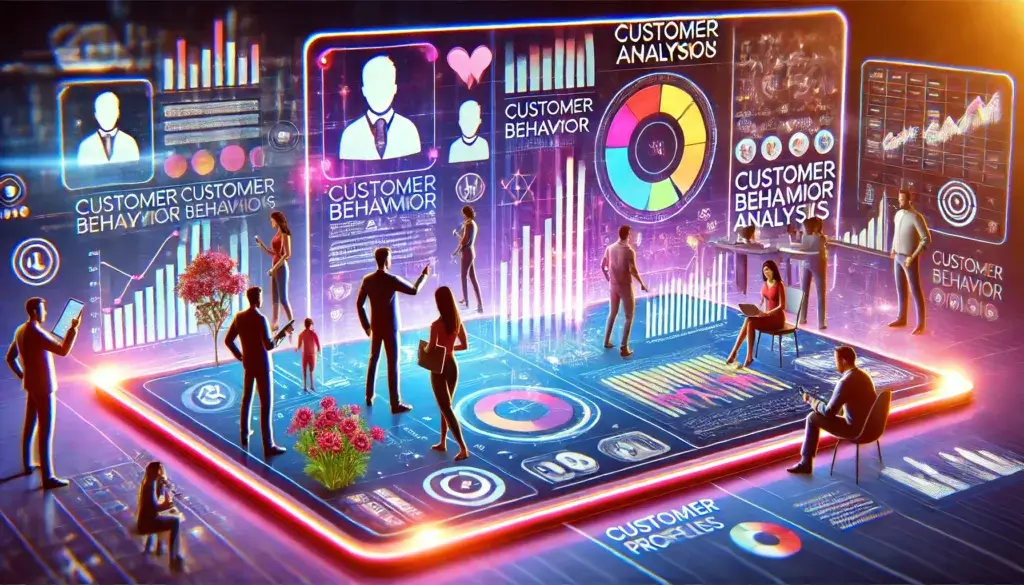
Drawing Actionable Insights from the Analysis
Data analysis isn’t just about numbers; it’s about uncovering stories and trends that can guide decision-making. Look for key takeaways that can be translated into specific actions. For example, if your analysis shows that certain products have consistently higher sales, you might consider increasing inventory for those items or promoting them more prominently.
Actionable insights should be clear and direct. Ask yourself: “What is this data telling me to do next?” The more specific your insights, the easier it will be to implement changes that make a difference.
Aligning Findings with Business Objectives
As you interpret your results, it’s essential to keep your business goals in mind. Insights are most valuable when they support your larger strategy. For example, if your goal is to increase customer retention, focus on insights related to repeat purchases, customer satisfaction, or loyalty programs.
Aligning findings with your objectives helps you stay on track and ensures that your analysis translates into meaningful actions. Every insight should feel like a step toward a bigger goal, helping your business stay focused and intentional.
Identifying Areas for Improvement and Opportunities
Interpreting data is also about spotting gaps and new possibilities. Look for areas where performance didn’t meet expectations, as these could represent opportunities for improvement. For instance, if a specific product or region is underperforming, you might investigate further to understand why—and explore ways to turn things around.
On the other hand, your data might reveal untapped opportunities. Perhaps there’s a growing interest in a particular product category, or maybe a specific demographic is buying more frequently than others. Identifying these opportunities allows you to make proactive decisions, such as expanding product lines or targeting new customer segments.
Step 7: Implementation and Monitoring
With valuable insights in hand, the final step is to put them into action. Implementation is where data analysis becomes truly impactful, transforming insights into concrete strategies that can improve sales, streamline operations, or enhance customer experiences. And once these changes are in place, ongoing monitoring ensures that your adjustments are on track and continue to drive results over time.
Developing Action Plans Based on Insights
Start by creating a clear action plan based on the insights you’ve gathered. This plan should outline specific steps, responsibilities, and timelines for each action you’re going to take. For example, if you discovered that a particular sales channel is performing well, your action plan might include steps to allocate more resources to that channel, refine your messaging, or invest in targeted advertising.
An effective action plan turns high-level insights into step-by-step instructions, making it easy for your team to follow through and work toward shared goals.
Implementing Changes in Sales Strategies or Operations
With your plan in place, it’s time to start making changes. Depending on your insights, this could mean adjusting sales tactics, updating your marketing approach, or even changing how you manage customer relationships. For example, if you identified a trend in customer preferences, you might update your product lineup or modify your pricing strategy to reflect those preferences.
It’s important to communicate these changes clearly with your team and ensure everyone understands the purpose and expected outcomes. Implementation is often a collaborative effort, so make sure each person involved knows their role and has the resources they need to make the transition smooth.
Establishing a System for Ongoing Monitoring and Analysis
Once your changes are in place, ongoing monitoring is essential to ensure they’re having the intended effect. Set up a system to track the key metrics related to your changes—whether it’s increased sales, improved customer satisfaction, or higher conversion rates. Tools like dashboards, monthly reports, or regular check-ins can help you stay informed and spot any early signs of success or areas needing further adjustment.
Continuous analysis allows you to refine your strategies over time, making small tweaks as needed to keep your business moving in the right direction. Think of monitoring as a feedback loop that helps you stay agile, adaptable, and focused on achieving long-term growth.
Tools and Software for Sales Data Analysis
With so many tools available for analyzing sales data, it’s easier than ever to transform raw numbers into actionable insights. The right software can help you track trends, monitor KPIs, and even forecast future sales, all while making data easy to visualize and understand. Choosing the right tool for your business is crucial, so here’s an overview of some popular options and what to consider when making your choice.
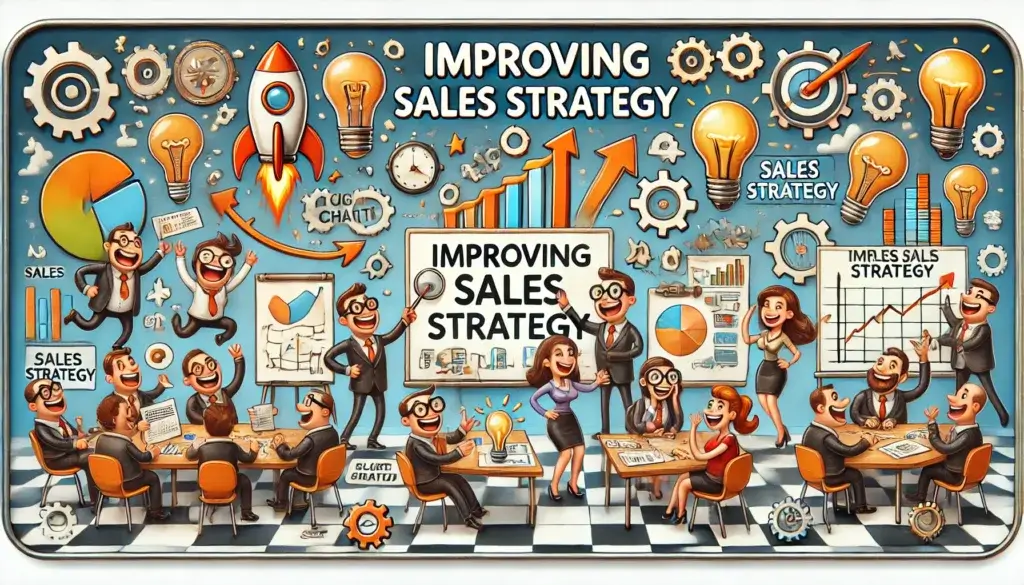
Overview of Popular Sales Analytics Tools
There’s a wide range of sales analytics tools on the market, each with its own strengths. Here are a few of the most popular ones:
- Salesforce: Known for its comprehensive CRM capabilities, Salesforce offers powerful analytics features that allow you to track customer interactions, forecast sales, and measure team performance. Its customizable dashboards make it a great choice for businesses that want detailed insights into customer behavior and sales processes.
- Looker: A favorite for data visualization, Looker helps you turn raw data into clear, interactive visuals that make complex trends easy to understand. It’s a particularly good choice for teams looking to build custom reports and dashboards that update in real-time.
- Power BI: Microsoft’s Power BI offers advanced analytics and visualization tools, making it ideal for businesses that need to handle large volumes of data from multiple sources. With integrations across Microsoft products, it’s a natural fit for companies already using tools like Excel or Azure.
- Google Data Studio: This free tool from Google is great for businesses on a budget. It allows you to pull data from various sources, including Google Analytics and Google Ads, making it a smart choice for teams focused on digital sales channels.
Each tool has its unique features, so the key is to choose one that matches your business needs and existing tech setup.
Criteria for Selecting the Right Tool for Your Business
With so many options, it’s essential to pick a tool that aligns with your specific needs. Here are some criteria to consider:
- Ease of Use: Choose a tool that suits your team’s level of technical expertise. Some tools offer user-friendly, drag-and-drop interfaces, while others require knowledge of SQL or coding to get the most out of them.
- Scalability: Consider your business’s future needs. As your company grows, you’ll want a tool that can handle increased data volume and more complex analyses without slowing down or requiring major upgrades.
- Customization Options: The ability to create custom reports, dashboards, and visualizations is a must for businesses with unique needs. Look for tools that allow you to tailor your analytics to match your specific KPIs and goals.
- Real-Time Data Access: If you need up-to-the-minute insights, choose a tool that supports real-time data processing. This can be crucial for fast-paced sales environments where timely information is key.
Selecting a tool that aligns with these criteria can save time and boost your team’s ability to make informed, data-driven decisions.
Integration Considerations with Existing Systems
A critical factor in choosing any new tool is how well it integrates with your existing systems. Here are a few things to keep in mind:
- CRM and ERP Integration: If you already use a CRM or ERP system, look for a tool that seamlessly connects with it. This integration can save time by automatically syncing customer data, sales records, and product information, ensuring that you’re always working with the latest data.
- Data Source Compatibility: Make sure the tool can pull data from all relevant sources, whether that’s an e-commerce platform, social media channels, or financial software. The ability to consolidate data from multiple places makes your analysis more comprehensive and reliable.
- APIs and Custom Connectors: Some tools offer APIs or allow for custom connectors, making it easier to integrate them into complex tech stacks. If your business relies on several different systems, having this flexibility is valuable for creating a unified view of your sales data.
Choosing a sales analytics tool with robust integration options helps streamline your workflows, reduces manual data entry, and allows you to see the bigger picture across all platforms. With the right tool in place, your sales data analysis becomes smoother, faster, and more insightful, helping your team make the most of every opportunity.
Case Studies and Examples
Seeing real-world examples of sales data analysis in action can make a huge difference in understanding the impact of data-driven decisions. By looking at how other businesses have successfully used sales data analysis, we can gather insights, learn from their challenges, and apply best practices to our own strategies. Let’s explore a few examples and the valuable lessons they offer.
Real-World Examples of Successful Sales Data Analysis
- Example 1: Retail Brand Optimizing Inventory with Predictive Analysis A retail brand used predictive analysis to better manage inventory levels across its stores. By analyzing past sales trends and seasonality, the brand was able to forecast demand more accurately. As a result, they optimized stock levels for high-demand items and reduced excess inventory for slower-moving products. This helped the brand cut down on storage costs and prevent stockouts, boosting both efficiency and customer satisfaction.
- Example 2: E-commerce Company Boosting Conversions with Customer Behavior Analysis An e-commerce company analyzed customer behavior data from their website, focusing on metrics like page visits, cart abandonment rates, and product views. By identifying points in the shopping journey where customers were dropping off, they made targeted changes to the website, such as streamlining the checkout process and personalizing product recommendations. These adjustments led to a noticeable increase in conversions and average order values, all thanks to insights gained from their sales data.
- Example 3: SaaS Business Improving Customer Retention with Cohort Analysis A SaaS company used cohort analysis to understand patterns in customer retention. By grouping customers based on when they signed up and tracking their engagement over time, the company discovered that users who engaged with certain features within the first week were more likely to renew their subscriptions. With this insight, they created a targeted onboarding experience to guide new users toward those features, which led to improved customer retention rates.
Lessons Learned and Best Practices
These examples highlight a few important lessons and best practices for successful sales data analysis:
- Start with a Clear Focus: Each of these companies had specific questions they wanted to answer—whether it was optimizing inventory, improving conversion rates, or boosting retention. Having a clear focus allowed them to direct their analysis efforts effectively and use their findings to solve real problems.
- Leverage Customer Behavior Data: Understanding customer behavior is often the key to uncovering valuable insights. Monitoring how customers interact with products, pages, or features helps identify opportunities to improve user experience, which can lead to higher engagement and sales.
- Act on Insights Quickly: Timely action is crucial. Once an analysis reveals actionable insights, implementing changes promptly can prevent issues or help you capture opportunities. These companies didn’t just identify problems; they quickly acted on the data, leading to impactful results.
- Use the Right Tools: Each business used tools suited to their specific needs, whether it was cohort analysis for retention or predictive modeling for inventory management. Choosing the right tools and techniques based on your goals and data types makes analysis more effective and your findings more useful.
Challenges and Solutions in Sales Data Analysis
Sales data analysis can be a powerful tool, but it’s not without its challenges. Many businesses encounter obstacles like data silos, inconsistent data, or even a lack of in-house expertise. The good news? These issues are common, and there are proven strategies for overcoming them. Let’s explore some of the most frequent hurdles and practical ways to tackle them.
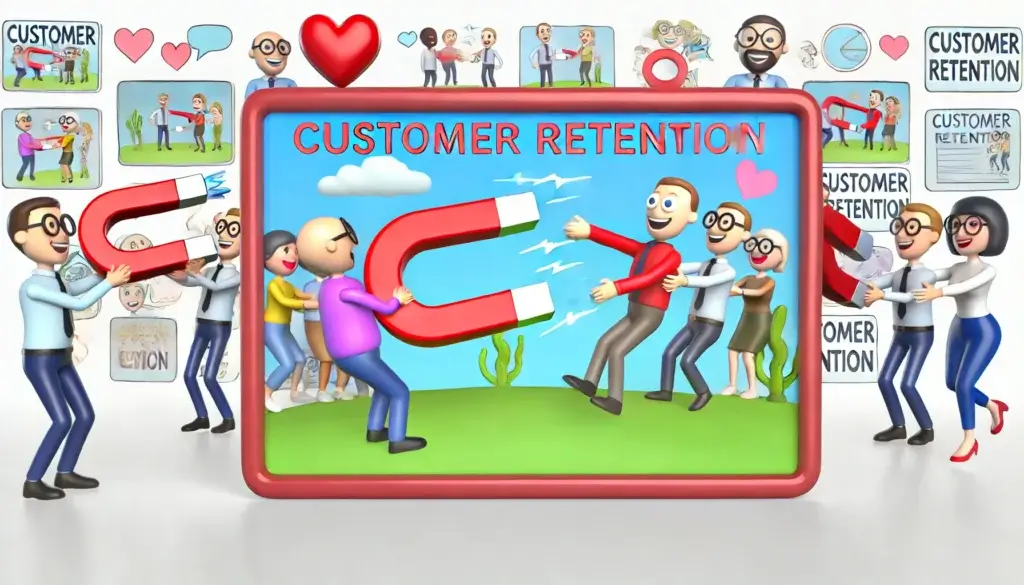
Common Obstacles
- Data Silos: Data silos occur when information is stored in isolated systems, making it difficult to get a complete view of your sales performance. For example, you may have customer data in one system, e-commerce data in another, and marketing metrics in yet another. This fragmentation can make it hard to pull everything together for a comprehensive analysis.
- Inconsistent Data: When data is collected from multiple sources, it often comes in different formats, which can lead to inconsistencies. Dates might be formatted differently, product names may not match exactly, or customer information may be incomplete. Inconsistent data can slow down the analysis process and lead to inaccuracies if not properly managed.
- Lack of Expertise: Not every business has a team of data analysts or experts on staff. Sales data analysis can require specialized skills, such as knowing how to clean and interpret data or being familiar with specific tools. Without this expertise, it can be challenging to make the most of your data.
Strategies to Overcome These Challenges
- Integrate Data Across Systems: To break down data silos, consider integrating your systems to create a single source of truth. Data integration tools like Zapier, MuleSoft, or custom APIs can help sync information across platforms, making it easier to access all your data in one place. Once your data is centralized, you’ll be able to conduct more comprehensive and insightful analyses.
- Standardize Data Collection and Formatting: Creating a consistent approach to data collection and formatting can minimize inconsistencies. Set guidelines for how data should be entered, such as standard date formats, naming conventions, and required fields. Regularly clean and update your data to ensure that everything aligns, making your analysis more accurate and less time-consuming.
- Invest in Training or Outsourcing: If expertise is a roadblock, investing in training can empower your team to handle basic data analysis tasks. Many tools offer user-friendly interfaces and tutorials that make it easier for non-experts to get started. Alternatively, consider outsourcing complex analyses to specialists or bringing in a consultant for a specific project. With the right support, even a small team can achieve meaningful insights.
Conclusion
Sales data analysis is more than just a numbers game—it’s a pathway to understanding your business, uncovering new opportunities, and making informed decisions that can drive growth. By following each step in this guide, from defining clear objectives to implementing insights and establishing a system for continuous monitoring, you’re setting up a process that brings data-driven clarity to your strategies.
Whether you’re aiming to optimize inventory, enhance customer engagement, or increase sales, effective data analysis gives you the tools to make these goals achievable. Embracing the right tools, visualizations, and best practices, while addressing common challenges like data silos and inconsistencies, can empower your team to work smarter and stay aligned with your business objectives.
As you move forward, remember that data analysis is an ongoing process. Consistently revisiting your data, refining your approach, and acting on fresh insights will keep your strategies adaptable and your business on track. In a world where data is one of the most valuable resources, leveraging sales analysis is a powerful way to stay competitive, make meaningful changes, and drive sustainable success.